SPCL_Bcast(COMM_WORLD)
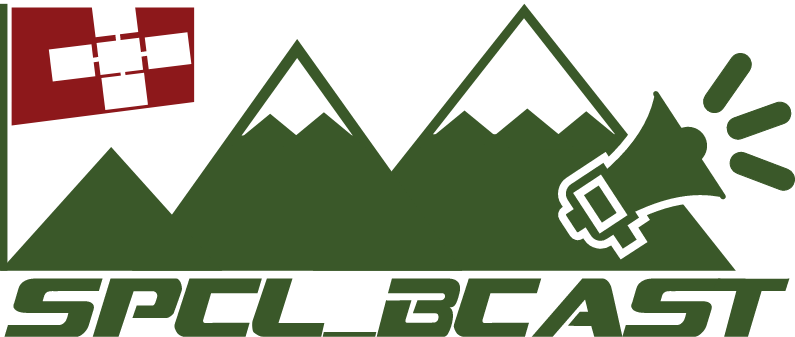
What: SPCL_Bcast
is an open, online seminar series that covers a broad range of topics around parallel and high-performance computing, scalable machine learning, and related areas.
Who: We invite top researchers and engineers from all over the world to speak.
Where: Anyone is welcome to join over Zoom! This link will always redirect to the right Zoom meeting. When possible, we make recordings available on our YouTube channel.
Old talks: See the SPCL_Bcast
archive.
Social media: Follow along with #spcl_bcast on Twitter!
When: Every two weeks on Thursdays, at 9AM or 6PM CET.
Programming Groq LPUs without IEEE Floating Point
Abstract: The IEEE standard has been a great advance in the early days of software. In these early days, the speed of software development was imperative. The Intel x86 instruction set became a standard as well as IEEE Floating point. Today, we have the first commodity computing application, the LLM, and others are rapidly following. In the commodity economy, efficiency and cost become the utmost imperative. As we are giving up on the x86 instruction set, we have to also consider custom number representations for each variable in our programs, opening the world of Physics and Computer Science to a new dimension in computing (as predicted in my talk at ETH in 2000). In this talk I will cover how to find the (locally) optimal range and precision for each variable, and how to optimally utilize custom precision arithmetic units in modern leading compute chips such as the Groq LPU.
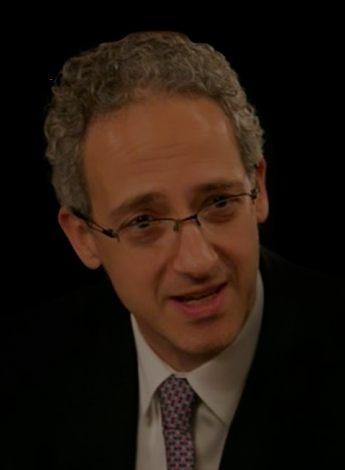
Homepage
Fugaku: The First 'Exascale' Supercomputer – Past, Present and Future
Abstract: Fugaku is the first 'exascale' supercomputer of the world, not due to its peak double precision flops, but rather, its demonstrated performance in real applications that were expected of exascale machines on their conceptions 10 years ago, as well as reaching actual exaflops in new breeds of benchmarks such as HPL-AI. But the importance of Fugaku is its "applications first" philosophy under which it was developed, and its resulting mission to be the centerpiece for rapid realization of the so-called Japanese 'Society 5.0' as defined by the Japanese S&T national policy. As such, Fugaku's immense power is directly applicable not only to traditional scientific simulation applications, but can be a target of Society 5.0 applications that encompasses conversion of HPC & AI & Big Data as well as Cyber (IDC & Network) vs. Physical (IoT) space, with immediate societal impact. In fact, Fugaku is already in partial operation a year ahead of schedule, primarily to obtain early Society 5.0 results including combatting COVID-19 as well as resolving other important societal issues. The talk will introduce how Fugaku had been conceived, analyzed, and built over the 10 year period, look at its current efforts regarding Society 5.0 and COVID, as well as touch upon our thoughts on the next generation machine, or "Fugaku NeXT".
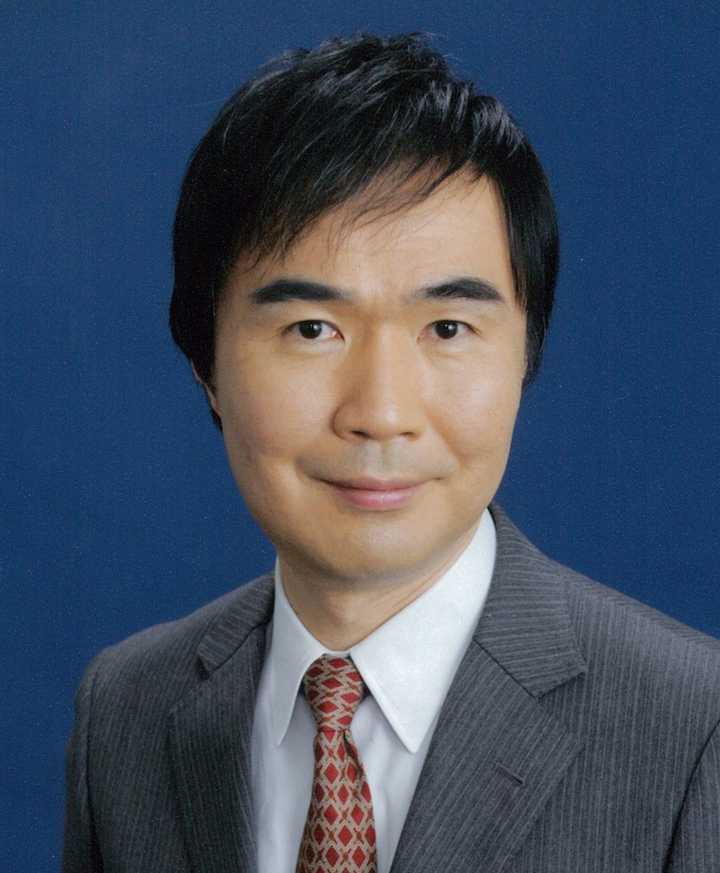
Homepage
A Paradigm Shift to Second Order Methods for Machine Learning
Abstract: The amount of compute needed to train modern NN architectures has been doubling every few months. With this trend, it is no longer possible to perform brute force hyperparameter tuning to train the model to good accuracy. However, first-order methods such as Stochastic Gradient Descent are quite sensitive to such hyperparameter tuning and can easily diverge for challenging problems. However, many of these problems can be addressed with second-order optimizers. In this direction, we introduce AdaHessian, a new stochastic optimization algorithm. AdaHessian directly incorporates approximate curvature information from the loss function, and it includes several novel performance-improving features, including: (i) a fast Hutchinson based method to approximate the curvature matrix with low computational overhead; and (ii) a spatial/temporal block diagonal averaging to smooth out variations of second-derivate over different parameters/iterations. Extensive tests on NLP, CV, and recommendation system tasks, show that AdaHessian achieves state-of-the-art results, with 10x less sensitivity to hyperparameter tuning as compared to ADAM.
In particular, we find that AdaHessian:
(i) outperforms AdamW for transformers by 0.13/0.33 BLEU score on IWSLT14/WMT14, 2.7/1.0 PPL on PTB/Wikitext-103;
(ii) outperforms AdamW for SqueezeBert by 0.41 points on GLUE;
(iii) achieves 1.45%/5.55% higher accuracy on ResNet32/ResNet18 on Cifar10/ImageNet as compared to Adam; and
(iv) achieves 0.032% better score than AdaGrad for DLRM on the Criteo Ad Kaggle dataset.
The cost per iteration of AdaHessian is comparable to first-order methods, and AdaHessian exhibits improved robustness towards variations in hyperparameter values.
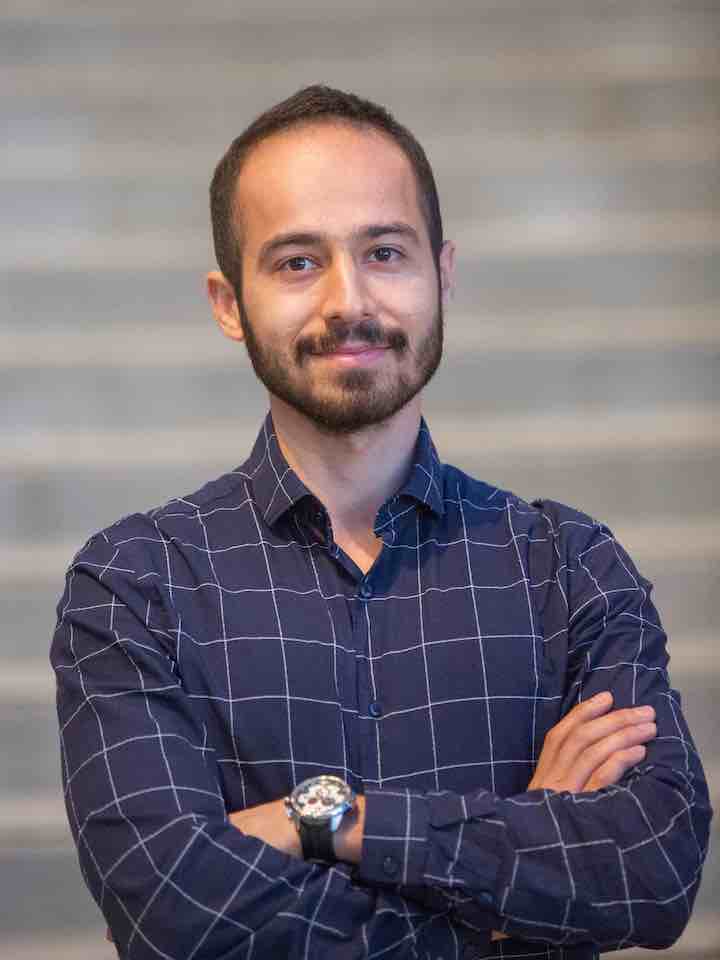
Homepage
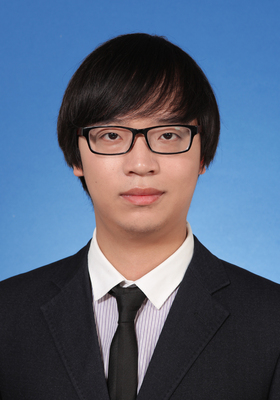
Homepage
Distributed Deep Learning with Second Order Information
Abstract: As the scale of deep neural networks continues to increase exponentially, distributed training is becoming an essential tool in deep learning. Especially in the context of un/semi/self-supervised pretraining, larger models tend to achieve much higher accuracy. This trend is especially clear in natural language processing, where the latest GPT-3 model has 175 billion parameters. The training of such models requires hybrid data+model-parallelism. In this talk, I will describe two of our recent efforts; 1) second-order optimization and 2) reducing memory footprint, in the context of large-scale distributed deep learning.
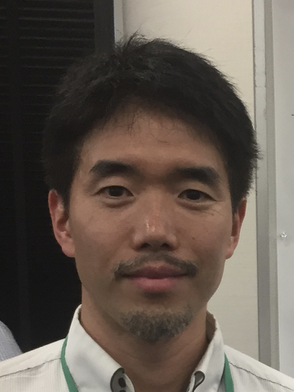
Homepage
High Performance Tensor Computations
Abstract: Tensor decompositions, contractions, and tensor networks are prevalent in applications ranging from data modeling to simulation of quantum systems. Numerical kernels within these methods present challenges associated with sparsity, symmetry, and other types of tensor structure. We describe recent innovations in algorithms for tensor contractions and tensor decompositions, which minimize costs and improve scalability. Further, we highlight new libraries for (1) automatic differentiation in the context of high-order tensor optimization, (2) efficient tensor decomposition, and (3) tensor network state simulation. These libraries all build on distributed tensor contraction kernels for sparse and dense tensors provided by the Cyclops library, enabling a shared ecosystem for applications of tensor computations.
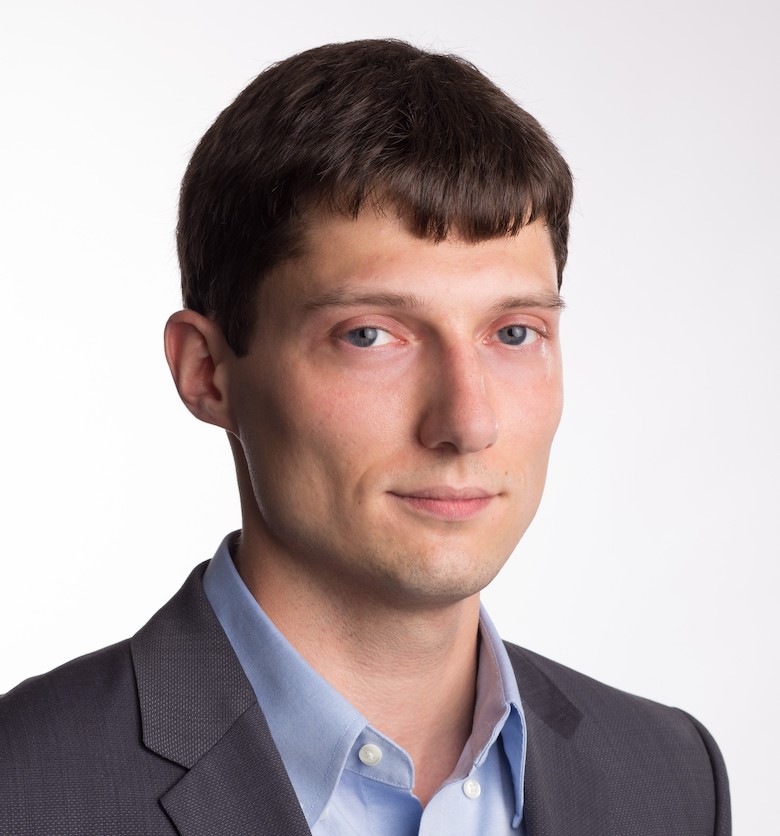
Homepage
Light-Weight Performance Analysis for Next-Generation HPC Systems
Abstract: Building efficient and scalable performance analysis and optimizing tools, for large-scale systems, is increasingly important both for the developers of parallel applications and the designers of next-generation HPC systems. However, conventional performance tools suffer from significant time/space overhead due to the ever-increasing problem size and system scale. On the other hand, the cost of source code analysis is independent of the problem size and system scale, making it very appealing for large-scale performance analysis. Inspired by this observation, we have designed a series of light-weight performance tools for HPC systems, such as memory access monitoring, performance variance detection, and communication compression. In this talk, I will share our expreience on building these tools through combining static analysis and runtime analysis and also point out the main challenges in this direction.
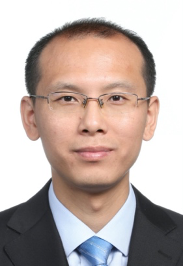
Homepage
Decomposing MPI Collectives for Exploiting Multi-lane Communication
Abstract: Many modern, high-performance systems increase the cumulated node-bandwidth by offering more than a single communication network and/or by having multiple connections to the network, such that a single processor-core cannot by itself saturate the off-node bandwidth. Efficient algorithms and implementations for collective operations as found in, e.g., MPI, must be explicitly designed for exploiting such multi-lane capabilities. We are interested in gauging to which extent this might be the case.
In the talk, I will illustrate how we systematically decompose the MPI collectives into similar operations that can execute concurrently on and exploit multiple network lanes. Our decomposition is applicable to all standard, regular MPI collectives, and our implementations' performance can be readily compared to the native collectives of any given MPI library. Contrary to expectation, our full-lane, performance guideline implementations in many cases show surprising performance improvements with different MPI libraries on different systems, indicating severe problems with native MPI library implementations. In many cases, our full-lane implementations are large factors faster than the corresponding library MPI collectives. The results indicate considerable room for improvement of the MPI collectives in current MPI libraries including a more efficient use of multi-lane capabilities.
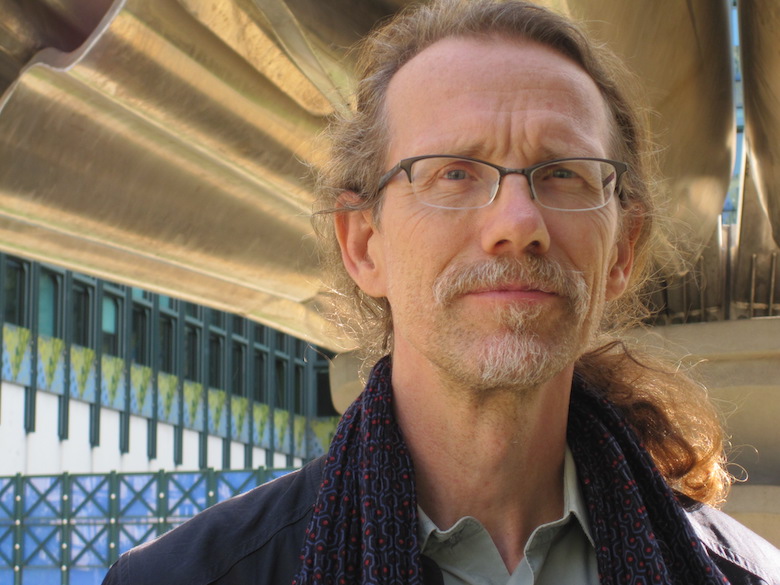
Homepage
Large Graph Processing on Heterogeneous Architectures: Systems, Applications and Beyond
Abstract: Graphs are de facto data structures for many data processing applications, and their volume is ever growing. Many graph processing tasks are computation intensive and/or memory intensive. Therefore, we have witnessed a significant amount of effort in accelerating graph processing tasks with heterogeneous architectures like GPUs, FPGAs and even ASICs. In this talk, we will first review the literatures of large graph processing systems on heterogeneous architectures. Next, we present our research efforts, and demonstrate the significant performance impact of hardware-software co-design on designing high performance graph computation systems and applications. Finally, we outline the research agenda on challenges and opportunities in the system and application development of future graph processing.
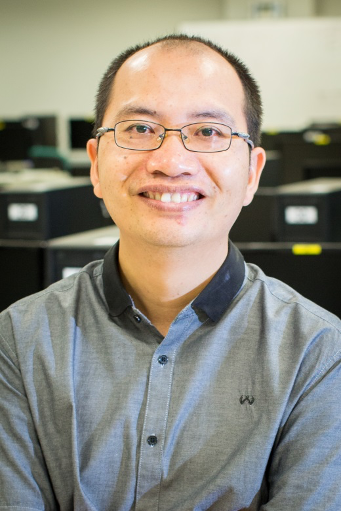
Homepage
Enabling Rapid COVID-19 Small Molecule Drug Design Through Scalable Deep Learning of Generative Models
Abstract: We improved the quality and reduced the time to produce machine-learned models for use in small molecule antiviral design. Our globally asynchronous multi-level parallel training approach strong scales to all of Sierra with up to 97.7% efficiency. We trained a novel, character-based Wasserstein autoencoder that produces a higher quality model trained on 1.613 billion compounds in 23 minutes while the previous state-of-the-art takes a day on 1 million compounds. Reducing training time from a day to minutes shifts the model creation bottleneck from computer job turnaround time to human innovation time. Our implementation achieves 318 PFLOPS for 17.1% of half-precision peak. We will incorporate this model into our molecular design loop, enabling the generation of more diverse compounds: searching for novel, candidate antiviral drugs improves and reduces the time to synthesize compounds to be tested in the lab.
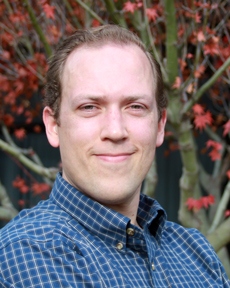
Homepage
Optimizing CESM-HR on Sunway TaihuLight and An Unprecedented Set of Multi-Century Simulations
Abstract: CESM is one of the very first and most complex scientific codes that gets migrated onto Sunway TaihuLight. Being a community code involving hundreds of different dynamic, physics, and chemistry processes, CESM brings severe challenges for the many-core architecture and the parrallel scale of Sunway TaihuLight. This talk summarizes our continuous effort on enabling efficient run of CESM on Sunway, starting from refactoring of CAM in 2015, redesigning of CAM in 2016 and 2017, and a collaborative effort starting in 2018 to enable highly efficient simulations of the high-resolution (25 km atmosphere and 10 km ocean) Community Earth System Model (CESM-HR) on Sunway Taihu-Light. The refactoring and optimizing efforts have improved the simulation speed of CESM-HR from 1 SYPD (simulation years per day) to 5 SYPD (with output disabled). Using CESM-HR, We manage to provide an unprecedented set of high-resolution climate simulations, consisting of a 500-year pre-industrial control simulation and a 250-year historical and future climate simulation from 1850 to 2100. Overall, high-resolution simulations show significant improvements in representing global mean temperature changes, seasonal cycle of sea-surface temperature and mixed layer depth, extreme events and in relationships between extreme events and climate modes.
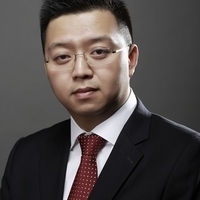
Evaluating modern programming models using the Parallel Research Kernels
Abstract: The Parallel Research Kernels were developed to support empirical studies of programming models in a variety of contexts without the porting effort required by proxy or mini-applications. I will describe the project and why it has been a useful tool in a variety of contexts and present some of our findings related to modern C++ parallelism for CPU and GPU architectures.
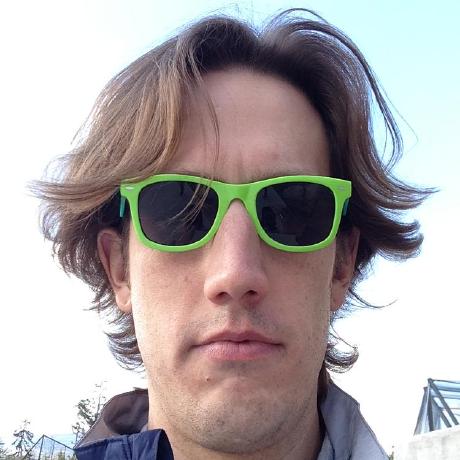
Homepage
High-Performance Sparse Tensor Operations in HiParTI Library
Abstract: This talk will present the recent development of HiParTI, a Hierarchical Parallel Tensor Infrastructure. I will emphasize on the element-wise sparse tensor contractions, commonly shown in quantum chemistry, physics, and others. We introduce three optimization techniques by using multi-dimensional, efficient hashtable representation for the accumulator and larger input tensor, and all-stage parallelization. Evaluating with 15 datasets, we obtain 28 - 576x speedup over the traditional sparse tensor contraction. With our proposed algorithm- and memory heterogeneity-aware data management, extra performance improvement is achieved on the heterogeneous memory with DRAM and Intel Optane DC Persistent Memory Module (PMM) over a state-of-the-art solutions.
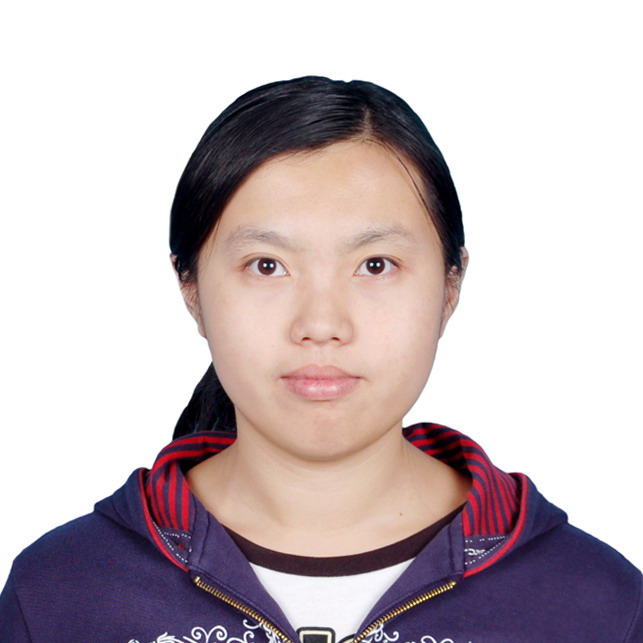
Homepage
HPHPC: High Productivity High Performance Computing with Legion and Legate
Abstract: This talk will describe the co-design and implementation of Legion and Legate, two programming systems that synergistically combine to provide to high productivity high performance computing ecosystem. In the first part of the talk, we'll introduce Legion, a task-based runtime system for supercomputers with a strong data model that enables a sophisticated dependence analysis. The second part of the talk will cover Legate, a framework for constructing drop-in replacements for popular Python libraries such as NumPy and Pandas on top of Legion. We'll show how using Legate and Legion together allows users to run unmodified Python programs at scale on hundreds of GPUs simply by changing a few import statements. We'll also discuss how the Legate framework makes it possible to compose such libraries even in distributed settings.
Homepage
Performance Engineering for Sparse Matrix-Vector Multiplication: Some new ideas for old problems
Abstract: The sparse matrix-vector multiplication (SpMV) kernel is a key performance component of numerous algorithms in computational science. Despite the kernel's apparent simplicity, the sparse and potentially irregular data access patterns of SpMV and its intrinsically low computational intensity haven been challenging the development of high-performance implementations over decades. Still these developments are rarely guided by appropriate performance models.
This talk will address the basic problem of understanding (i.e., modelling) and improving the computational intensity of SpMV kernels with a focus on symmetric matrices. Using a recursive algebraic coloring (RACE) of the underlying undirected graph, a node-level parallel symmetric SpMV implementation is developed which increases the computational intensity and the performance for a large general set of matrices by a factor of up to 2x. The same idea is then applied to accelerate the computation sparse matrix powers via cache blocking.
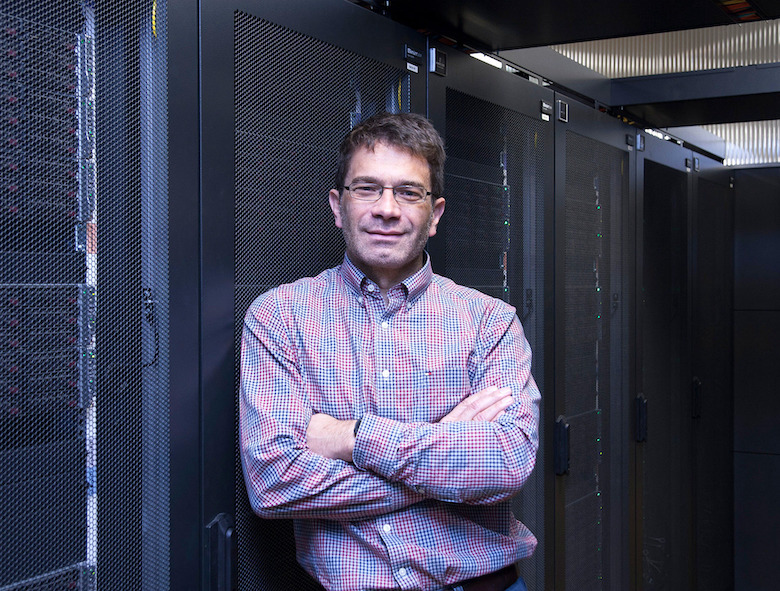
Gerhard Wellein has more than twenty years of experience in teaching HPC techniques to students and scientists from computational science and engineering, is an external trainer in the Partnership for Advanced Computing in Europe (PRACE) and received the "2011 Informatics Europe Curriculum Best Practices Award" (together with Jan Treibig and Georg Hager) for outstanding teaching contributions. His research interests focus on performance modelling and performance engineering, architecture-specific code optimization, novel parallelization approaches and hardware-efficient building blocks for sparse linear algebra and stencil solvers. He has been conducting and leading numerous HPC projects including the German Japanese project "Equipping Sparse Solvers for Exascale" (ESSEX) within the DFG priority program SPPEXA ("Software for Exascale Computing").
Homepage
Cloud-Scale Inference on FPGAs at Microsoft Bing
Abstract: Microsoft's Project Catapult began nearly a decade ago, leading to the widespread deployment of FPGAs in Microsoft's data centers for application and network acceleration. Project Brainwave began five years later, applying those FPGAs to accelerate DNN inference for Bing and later other Microsoft cloud services. FPGA flexibility has enabled the Brainwave architecture to evolve rapidly, keeping pace with rapid developments in the DNN model space. The low cost of updating FPGA-based designs also enables greater risk taking, facilitating innovations such as our Microsoft Floating Point (MSFP) data format. FPGAs with hardened support for MSFP will provide a new level of performance for Brainwave. These AI-optimized FPGAs also introduce a new point in the hardware spectrum between general-purpose devices and domain-specific accelerators. Going forward, a key challenge for accelerator architects will be finding the right balance between hardware specialization, hardware configurability, and software programmability.
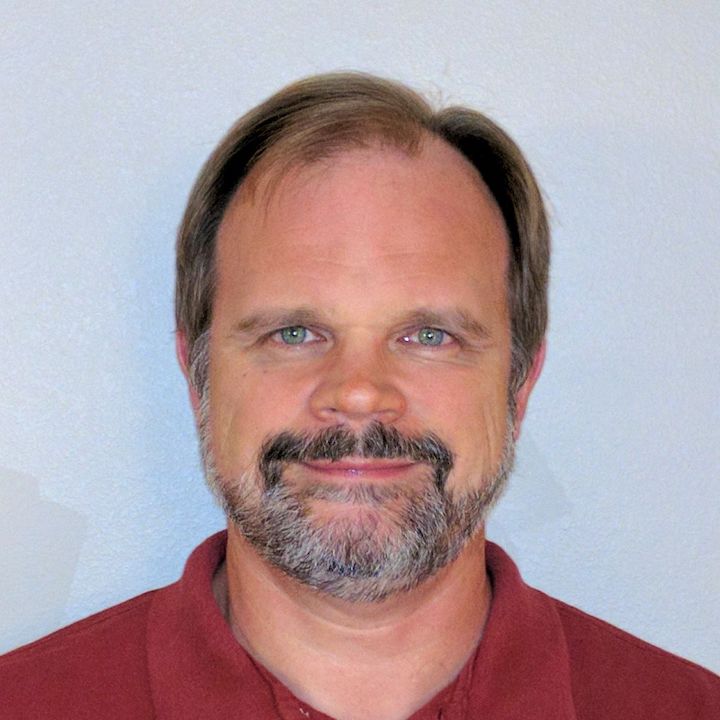
Homepage
Inspecting Irregular Computation Patterns to Generate Fast Code
Abstract: Sparse matrix methods are at the heart of many scientific computations and data analytics codes. Sparse matrix kernels often dominate the overall execution time of many simulations. Further, the indirection from indexing and looping over the nonzero elements of a sparse data structure often limits the optimization of such codes. In this talk, I will introduce Sympiler, a domain-specific code generator that transforms computation patterns in sparse matrix methods for high-performance. Specifically, I will show how decoupling symbolic analysis from numerical manipulation will enable the automatic optimization of sparse codes. I will also demonstrate the application of symbolic analysis in accelerating quadratic program solvers.
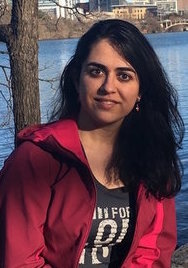
Homepage
Transferable Deep Learning Surrogates for Solving PDEs
Abstract: Partial differential equations (PDEs) are ubiquitous in science and engineering to model physical phenomena. Notable PDEs are the Laplace and Navier-Stokes equations with numerous applications in fluid dynamics, electrostatics, and steady-state heat transfer. Solving such PDEs relies on numerical methods such as finite element, finite difference, and finite volume. While these methods are extremely powerful, they are also computationally expensive. Despite widespread efforts to improve the performance and scalability of solving these systems of PDEs, several problems remain intractable.
In this talk, we'll explore the potential of deep learning (DL)-based surrogates to both augment and replace numerical simulations. In the first part of the talk, we'll present two frameworks -- CFDNet and SURFNet, that couple simulations with a convolutional neural network to accelerate the convergence of the overall scheme without relaxing the convergence constraints of the physics solver. The second part of the talk will introduce another novel framework that leverages DL to build a transferable deep neural network surrogate that solves PDEs in unseen domains with arbitrary boundary conditions. We'll show that a DL model trained only once can be used forever without re-training to solve PDEs in large and complex domains with unseen sizes, shapes, and boundary conditions. Compared with the state-of-the-art physics-informed neural networks for solving PDEs, we demonstrate 1-3 orders of magnitude speedups while achieving comparable or better accuracy.
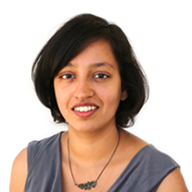
Homepage
Exploring Tools & Techniques for the Frontier Exascale System: Challenges vs Opportunities
Abstract: PIConGPU, an extremely scalable, heterogeneous, fully relativistic particle-in-cell (PIC) C++ code provides a modern simulation framework for laser-plasma physics and laser-matter interactions suitable for production-quality runs on large scale systems. This plasma physics application is fueled by alpaka abstraction library and incorporates openPMD-API enabling I/O libraries such as ADIOS2. Among many supercomputing systems, PIConGPU has been running on ORNL's Titan, Summit and is expected to run on the Exascale system, Frontier, that is being built as we speak. This talk will discuss some of the challenges, opportunities and potential solutions with respect to maintaining a performant portable code while migrating the same to Frontier.
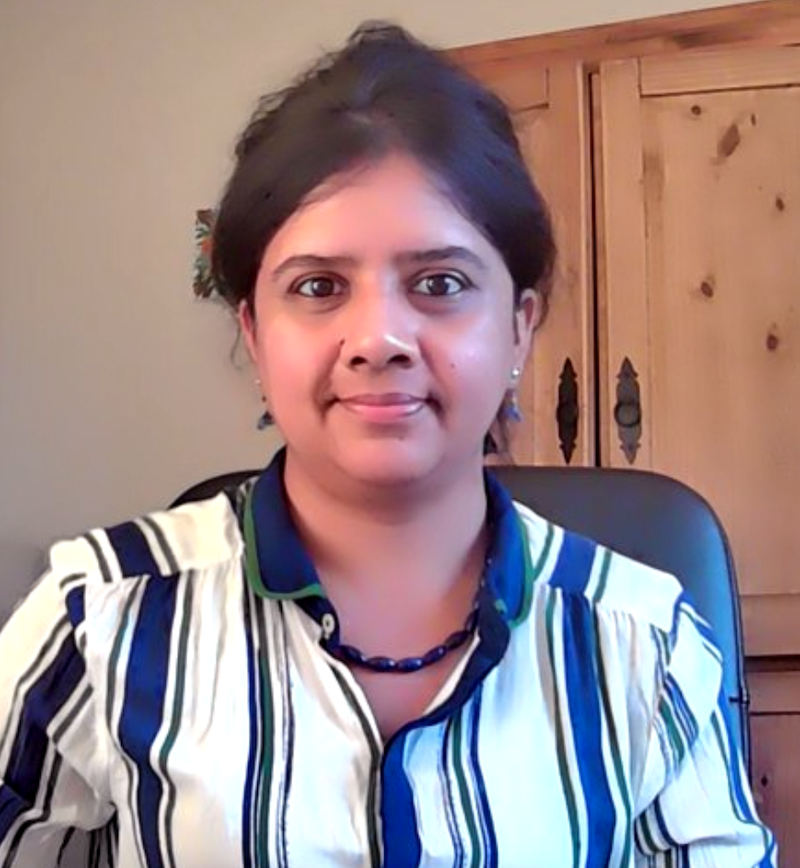
Homepage
High Performance Computing: Beyond Moore's Law
Abstract: Supercomputer performance now exceeds that of the earliest computers by thirteen orders of magnitude, yet science still needs more than they provide. But with Dennard scaling and Moore's Law ending even as AI and HPC demand continued growth. Demand engenders supply, and ways to prolong the growth in supercomputing performance are at hand or on the horizon. Architectural specialization has returned, after a loss of system diversity in the Moore's law era; it provides a significant boost for computational science. And at the hardware level, the development by Cerebras of a viable wafer-scale compute platform has important ramifications. Other long-term possibilities, notably quantum computing, may eventually play a role.
Why wafer-scale? Real achieved performance in supercomputers (as opposed to the peak speed) is limited by the bandwidth and latency barriers --- memory and communication walls --- that impose delay when off-processor-chip data is needed, and it is needed all the time. By changing the scale of the chip by two orders of magnitude, we can pack a small, powerful, mini-supercomputer on one piece of silicon, and eliminate much of the off-chip traffic for applications that can fit in the available memory. The elimination of most off-chip communication also cuts the power per unit performance, a key parameter when total system power is capped, as it usually is.
Cerebras overcame technical problems concerning yield, packaging, cooling, and delivery of electrical power in order to make wafer-scale computing viable. The Cerebras second generation wafer has over 800,000 identical processing elements architected with features that support sparsity and power-efficient performance. For ML, algorithmic innovations such as conditional computations and model and data sparsity promise significant savings in memory and computation while preserving model capacity. Flexible hardware rather than dense matrix multiply is required to best exploit these algorithmic innovations. We will discuss the aspects of the architecture that meet that requirement.
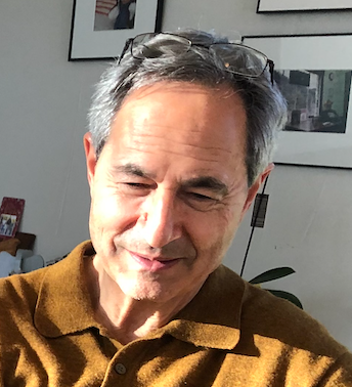
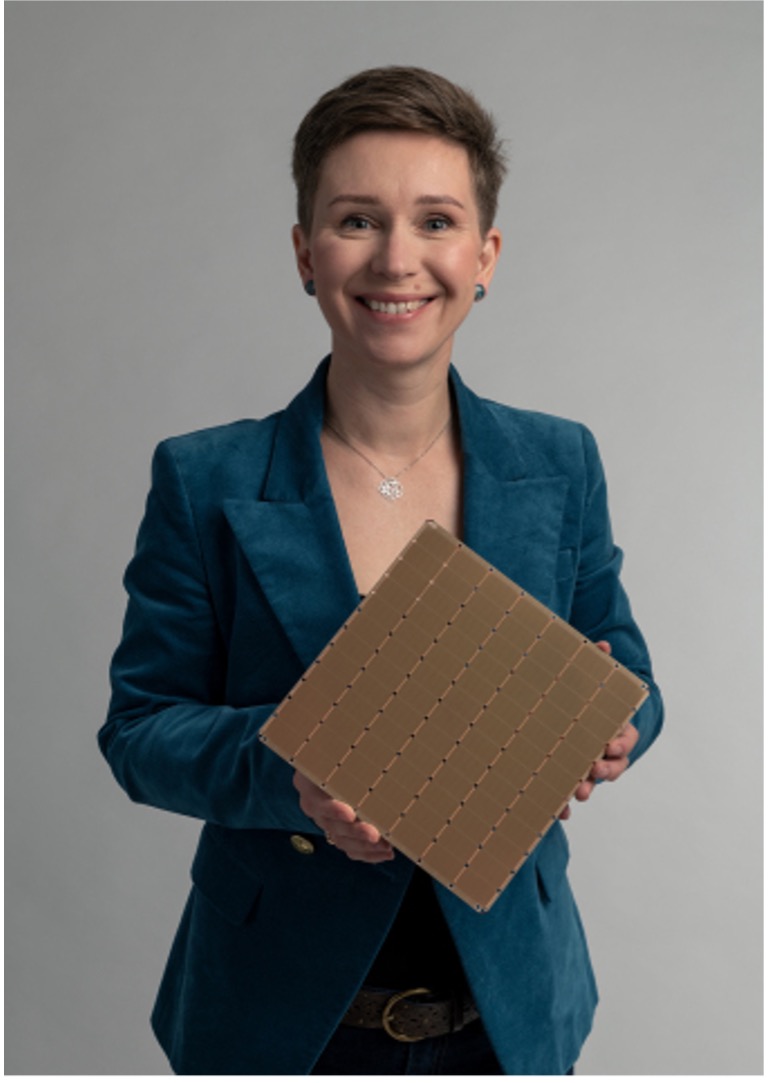
Homepage
Heterogeneous System Architectures: A Strategy to Use Diverse Components
Abstract: Current system architectures rely on a simple approach: one compute node design that is used across the entire system. This approach only supports heterogeneity at the node level. Compute nodes may involve a variety of devices but the system is otherwise homogeneous. This design simplifies scheduling applications and provides consistent expectations for the hardware that a job can exploit but often results in poor utilization of components. The wide range of emerging devices for AI and other domains necessitates a more heterogeneous system architecture that varies the compute node (or volume) types within a single job.
Lawrence Livermore National Laboratory (LLNL) is currently exploring such heterogeneous system architectures. These explorations include the use of novel hardware to accelerate AI models within larger applications and initial software solutions to overcome the challenges posed by heterogeneous system architectures. This talk will present a sampling of the novel software solutions that enable the heterogeneous system architecture as well as the systems that LLNL has currently deployed.
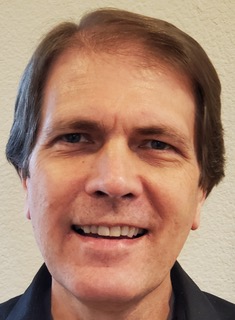
Homepage
TinyML and Efficient Deep Learning
Abstract: Today's AI is too big. Deep neural networks demand extraordinary levels of data and computation, and therefore power, for training and inference. This severely limits the practical deployment of AI in edge devices. We aim to improve the efficiency of neural network design. First, I'll present MCUNet that brings deep learning to IoT devices. MCUNet is a framework that jointly designs the efficient neural architecture (TinyNAS) and the light-weight inference engine (TinyEngine), enabling ImageNet-scale inference on micro-controllers that have only 1MB of Flash. Next I will introduce Once-for-All Network, an efficient neural architecture search approach, that can elastically grow and shrink the model capacity according to the target hardware resource and latency constraints. From inference to training, I'll present TinyTL that enables tiny transfer learning on-device, reducing the memory footprint by 7-13x. Finally, I will describe data-efficient GAN training techniques that can generate photo-realistic images using only 100 images, which used to require tens of thousands of images. We hope such TinyML techniques can make AI greener, faster, more efficient and more sustainable.
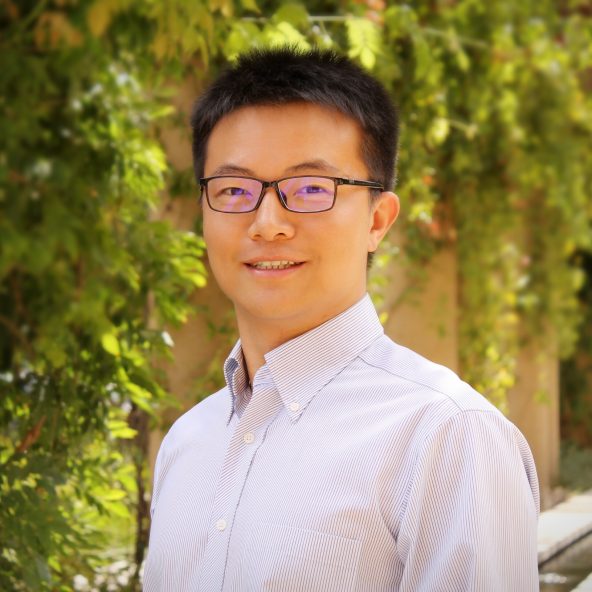
Homepage
Optimization of Data Movement for Convolutional Neural Networks
Abstract: Convolutional Neural Networks (CNNs) are central to Deep Learning. The optimization of CNNs has therefore received significant attention. Minimizing data movement is critical to performance optimization. This talk will address the minimization of data movement for CNNs in two scenarios. In the first part of the talk, the optimization of tile loop permutations and tile size selection will be discussed for executing CNNs on multicore CPUs. Most efforts on optimization of tiling for CNNs have either used heuristics or limited search over the huge design space. We show that a comprehensive design space exploration is feasible via analytical modeling. In the second part of the talk, communication minimization for executing CNNs on distributed systems will be discussed.
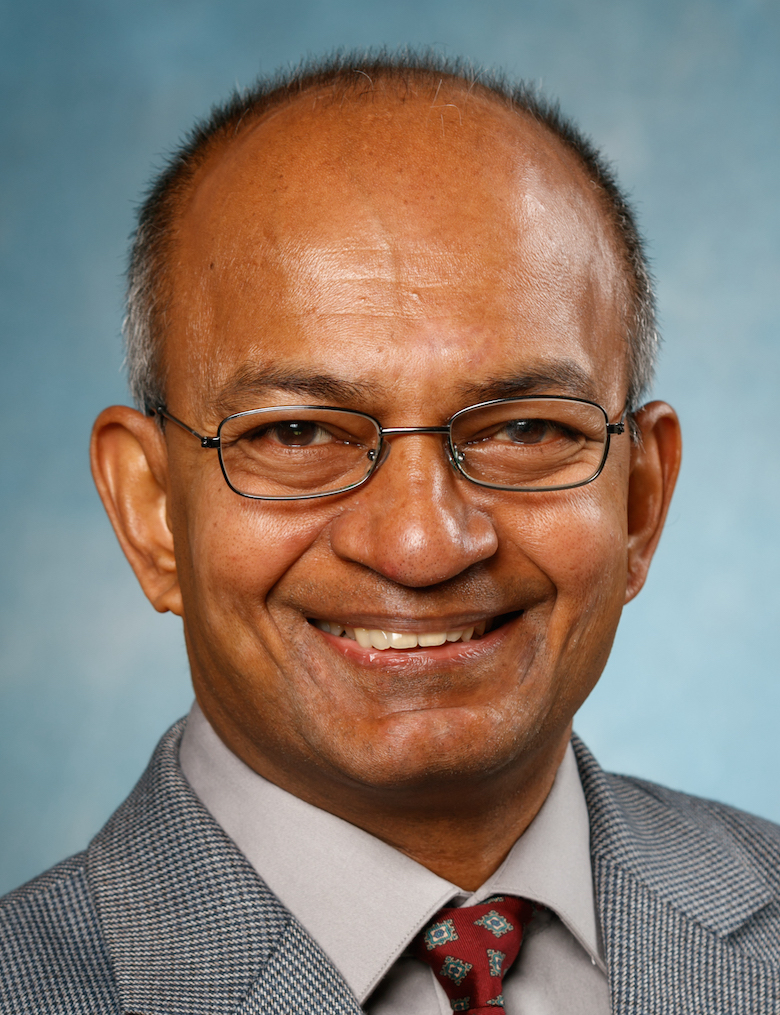
Homepage
Research with AIEngine and MLIR
Abstract: The Xilinx Versal devices include an array of AIEngine Vector-VLIW processor cores suitable for Machine Learning and DSP processing tasks. This talk will provide an overview of AIEngine-based devices and discuss how they are programmed. The talk will also present recent work to build open source tools for these devices based on MLIR to support a wide variety of high-level programming models.
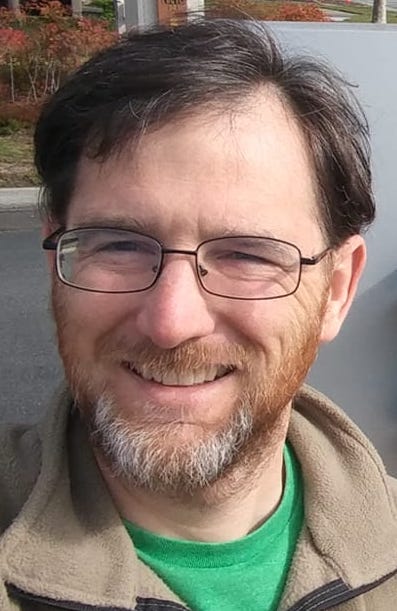
Homepage
Towards Next-Generation Numerical Methods with Physics-Informed Neural Networks
Abstract: Physics-Informed Neural Networks (PINNs) have recently emerged as a powerful tool for solving scientific computing problems. PINNs can be effectively used for developing surrogate models, completing data assimilation and uncertainty quantification tasks, and solving ill-defined problems, e.g., problems without boundary conditions or a closure equation. An additional application of PINNs is a central topic for scientific computing: the development of numerical solvers of Partial Differential Equations (PDE). While the accuracy and performance of PINNs for solving PDEs directly are still relatively low compared to traditional numerical solvers, combining traditional methods and PINNs opens up the possibility of designing new hybrid numerical methods. This talk introduces how PINNs work, emphasizing the relation between PINN components and main ideas with classical numerical methods, such as Finite Element Methods, Krylov solvers, and quasi-MonteCarlo techniques. I present PINNs' features that make them amenable to use in combination with traditional solvers. I then outline opportunities for developing a new class of numerical methods combining classical and neural network solvers, providing results from initial experiments.
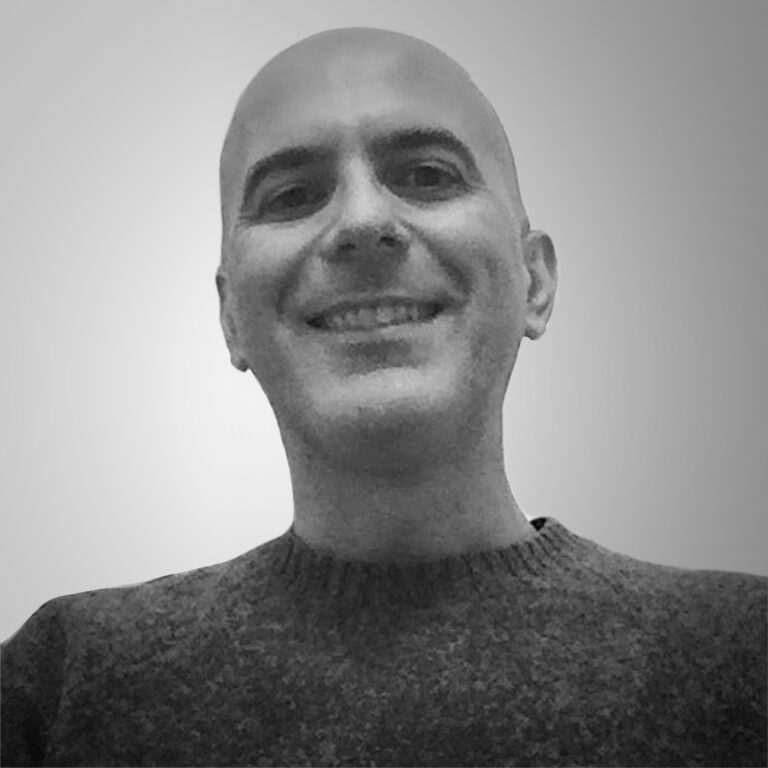
Parallel Sparse Matrix Algorithms for Data Analysis and Machine Learning
Abstract: In addition to the traditional theory and experimental pillars of science, we are witnessing the emergence of three additional pillars, which are simulation, data analysis, and machine learning. All three recent pillars of science rely on computing but in different ways. Matrices, and sparse matrices in particular, play an outsized role in all three computing related pillars of science, which will be the topic of my talk.
I will first highlight some of the emerging use cases of sparse matrices in data analysis and machine learning. These include graph computations, graph representation learning, and computational biology. The rest of my talk will focus on new parallel algorithms for such modern computations on sparse matrices. These include the use of "masking" for filtering out undesired output entries in sparse-times-sparse and dense-times-dense matrix multiplication, new distributed-memory algorithms for sparse matrix times tall-skinny dense matrix multiplication, combinations of these algorithms, and subroutines of them.
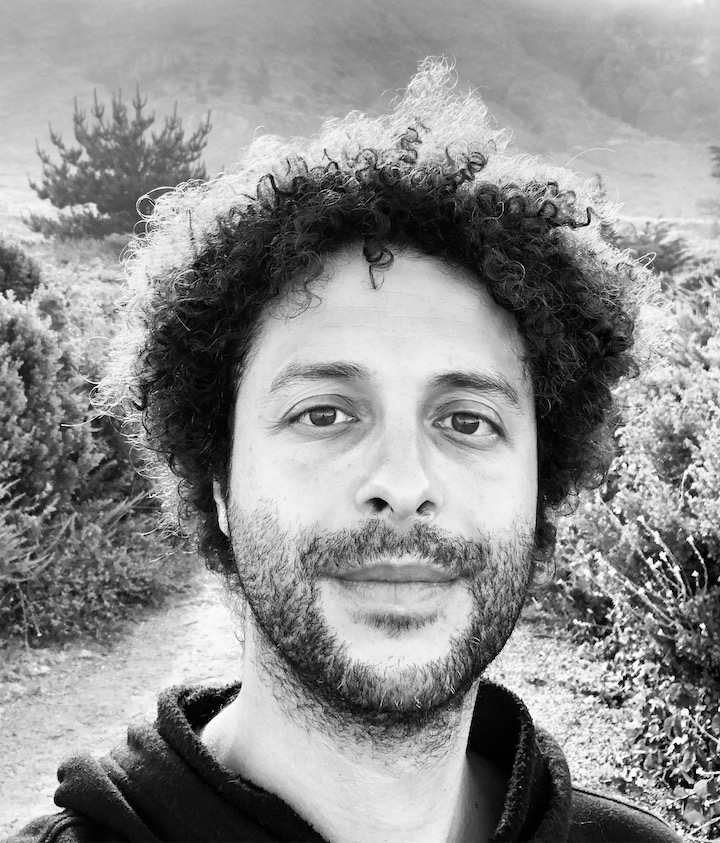
Homepage
Building Digital Twins of the Earth for NVIDIA's Earth-2 Initiative
Abstract: NVIDIA is committed to helping address climate change. Recently our CEO announced the Earth-2 initiative, which aims to build digital twins of the Earth and a dedicated supercomputer, E-2, to power them. Two central goals of this initiative are to predict the disastrous impacts of climate change well in advance and to help develop strategies to mitigate and adapt to change.
Here we present our work on an AI weather forecast surrogate trained on ECMWF's ERA5 reanalysis dataset. The model, called FourCastNet, employs a patch-based Vision-Transformer with a Fourier Neural Operator mixer. FourCastNet produces short to medium range weather predictions of about two dozen physical fields at 25-km resolution that exceed the quality of all related deep learning-based techniques to date. FourCastNet is capable of accurately forecasting fast timescale variables such as the surface wind speed, precipitation, and atmospheric water vapor with important implications for wind energy resource planning, predicting extreme weather events such as tropical cyclones and atmospheric rivers, as well as extreme precipitation. We compare the forecast skill of FourCastNet with archived operational IFS model forecasts and find that the forecast skill of our purely data-driven model is remarkably close to that of the IFS model for forecast lead times of up to 8 days. Furthermore, it can produce a 10-day forecast in a fraction of a second on a single GPU.
The enormous speed and high accuracy of FourCastNet provides at least three major advantages over traditional forecasts: (i) real-time user interactivity and analysis; (ii) the potential for large forecast ensembles; and (iii) the ability to combine fast surrogates to form new coupled systems. Large ensembles can capture rare but highly impactful extreme weather events and better quantify the uncertainty of such events by providing more accurate statistics. The figure below shows results from FourCastNet in NVIDIA's interactive Omniverse environment. On the left we show atmospheric rivers making landfall in California in Feb, 2017. On the right is a forecast of hurricane Matthew from Sept 2016. By plugging AI surrogates into Omniverse, users can generate, visualize, and explore potential weather outcomes interactively.
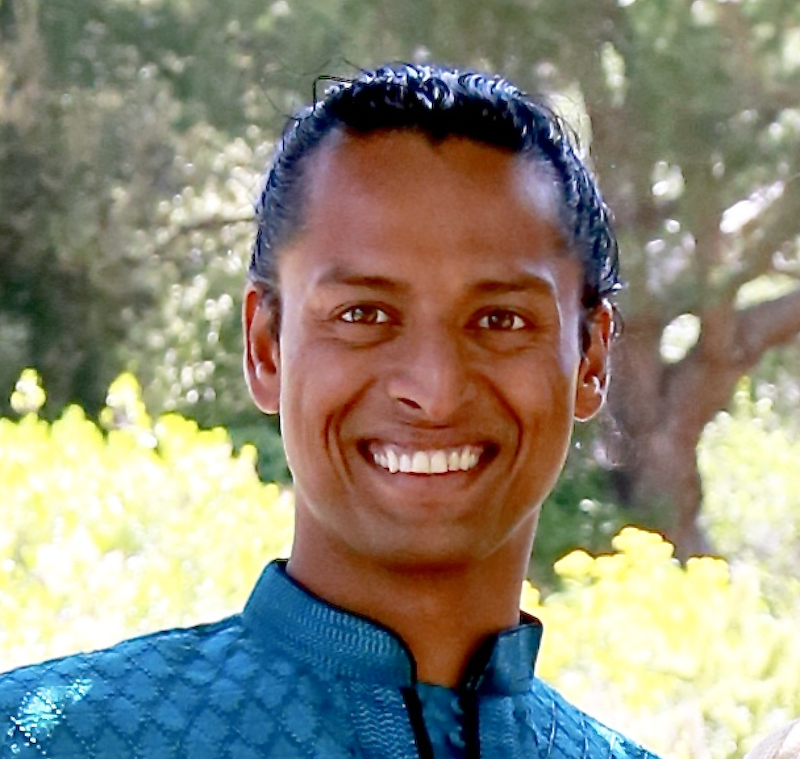
Language and Compiler Research for Heterogeneous Emerging Computing Systems
Abstract: Programming heterogeneous computing systems is still a daunting task that will become even more challenging with the advent of emerging, non Von-Neumann computer architectures. The so-called golden age of computer architecture thus must be accompanied by a, hopefully, golden age of research in compilers and programming languages. This talk discusses research along two fronts, namely, (1) on domain specific languages (DSLs) to hide complexity from non-expert programmers while passing richer information to compilers, and (2) on understanding the fundamental changes in emerging computing paradigms and their consequences for compilers. Concretely, we will talk about DSLs for physics simulations, compute-in-memory with emerging technologies, and current efforts in unifying intermediate representations with the MLIR compiler framework.
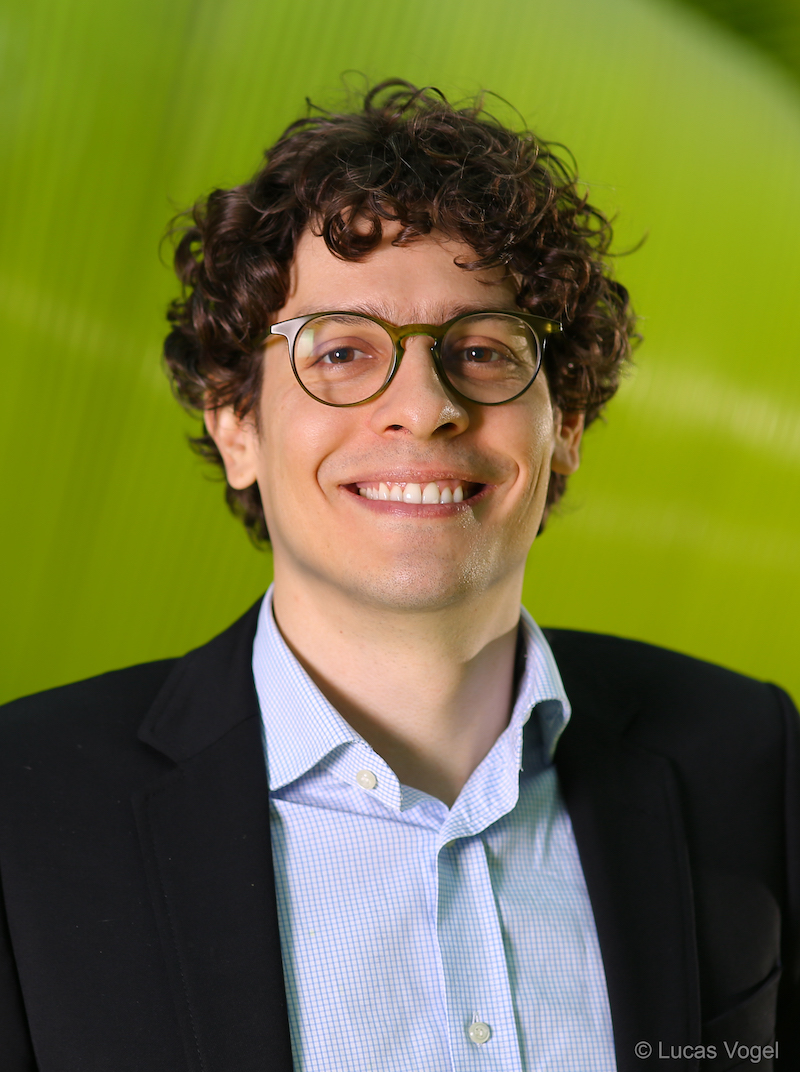
Homepage
Challenges of Scaling Deep Learning on HPC Systems
Abstract: Machine learning, and training deep learning in specific, are becoming one of the main workloads running on HPC systems. More so, the scientific computing community is increasingly adopting modern deep learning approaches to their workflows. When HPC practitioners attempt to scale a typical HPC workload, they are mostly challenged by a particular bottleneck. Scaling deep learning, on the other hand, can be challenged by different bottlenecks: memory capacity, communication, I/O, compute etc. In this talk we give an overview of the bottlenecks in scaling deep learning, and highlight efforts in addressing some of those bottlenecks: memory capacity and I/O.
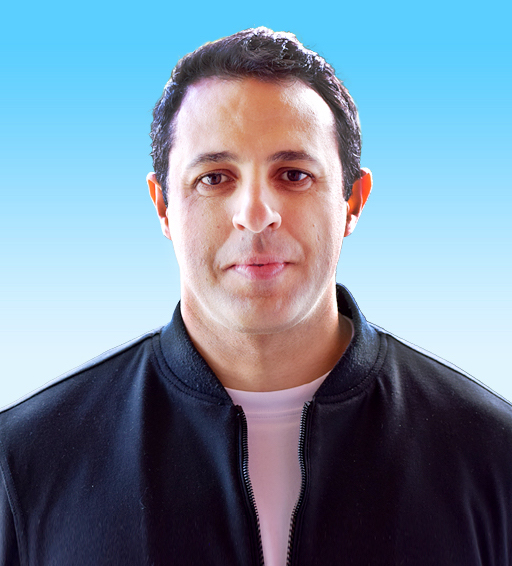
Homepage
Co-Optimization of Computation and Data Layout to Optimize Data Movement
Abstract: Code generation and optimization for the diversity of current and future architectures must focus on reducing data movement to achieve high performance. How data is laid out in memory, and representations that compress data (e.g., reduced floating point precision) have a profound impact on data movement. Moreover, the cost of data movement in a program is architecture-specific, and consequently, optimizing data layout and data representation must be performed by a compiler once the target architecture is known. With this context in mind, this talk will provide examples of data layout and data representation optimizations, and call for integrating these data properties into code generation and optimization systems.
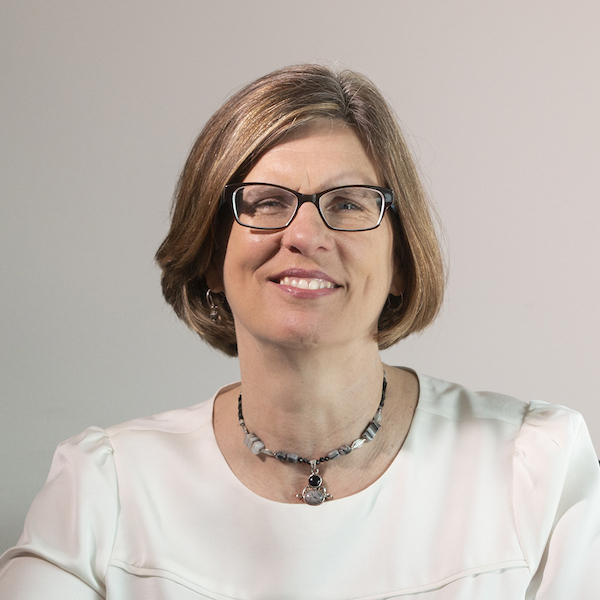
Homepage
Automating Distributed Heterogeneous Computing for Domain Experts
Abstract: Multiple simultaneous disruptions are currently under way in both hardware and software, as we consider the implications for future HPC systems. In hardware, "extreme heterogeneity" has become critical to sustaining cost and performance improvements after Moore's Law, but poses significant productivity challenges for developers. In software, the rise of large-scale AI and data analytics applications is being driven by domain experts from diverse backgrounds who demand the programmability that they have come to expect from high-level languages like Python. While current foundations for programming models, compilers, runtime systems, and debuggers have served us well for many decades, we now see signs of their limitations in the face of these disruptions. This talk makes a case for new approaches to enable productivity and programmability of future HPC systems for domain experts, and discusses recent approaches being explored in the Habanero Extreme Scale Software Research Laboratory. Preliminary results will be shared for the new compiler and runtime techniques being explored in our laboratory, including how we propose to respond to the challenge of automating distributed heterogeneous computing for Python-level domain experts.
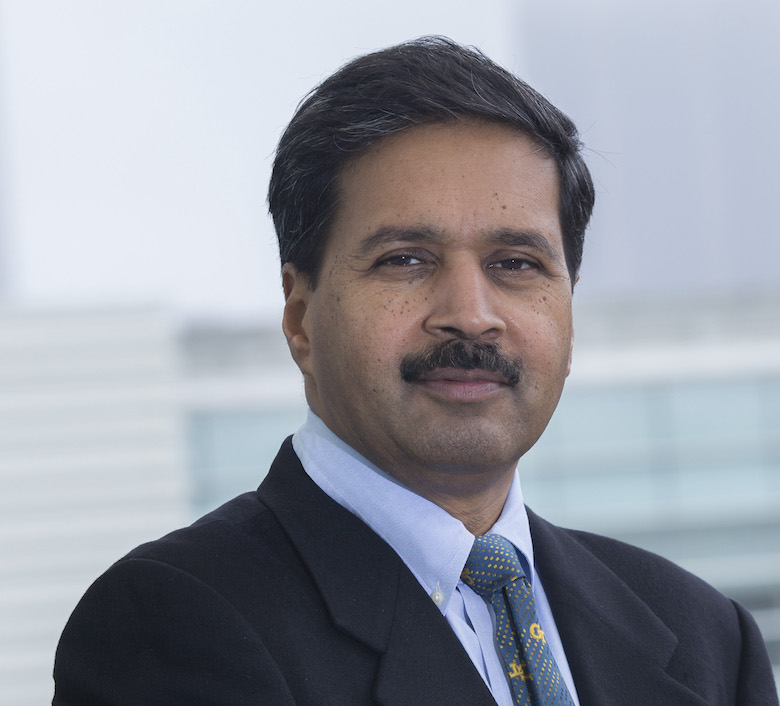
Sarkar started his career in IBM Research after obtaining his Ph.D. from Stanford University, supervised by John Hennessy. His research projects at IBM include the PTRAN automatic parallelization system led by Fran Allen, the ASTI optimizer for IBM's XL Fortran product compilers, the open-source Jikes Research Virtual Machine for the Java language, and the X10 programming language developed in the DARPA HPCS program. He was a member of the IBM Academy of Technology during 1995-2007, and Senior Manager of the Programming Technologies Department at IBM Research during 2000-2007. After moving to academia, Sarkar has mentored over 30 Ph.D. students and postdoctoral researchers in the Habanero Extreme Scale Software Research Laboratory, first at Rice University since 2007, and now at Georgia Tech since 2017. Researchers in his lab have developed the Habanero-C/C++ and Habanero-Java programming systems for parallel, heterogeneous, and distributed platforms. While at Rice, Sarkar was the E.D. Butcher Chair in Engineering, served as Chair of the Department of Computer Science, created a new sophomore-level course on the fundamentals of parallel programming, as well as a three-course Coursera specialization on parallel, concurrent, and distributed programming.
Sarkar is an ACM Fellow and an IEEE Fellow. He has been serving as a member of the US Department of Energy's Advanced Scientific Computing Advisory Committee (ASCAC) since 2009, and is currently co-chair of the recently created CRA-Industry committee (after serving on the CRA Board for seven years). Sarkar is also the recipient of the 2020 ACM-IEEE CS Ken Kennedy Award.
Homepage
Self-Adjusting Networks
Abstract: The bandwidth and latency requirements of modern datacenter applications have led researchers to propose various datacenter topology designs using static, dynamic demand-oblivious (rotor), and/or dynamic demand-aware switches. However, given the diverse nature of datacenter traffic, there is little consensus about how these designs would fare against each other. In this talk, I will present the vision of self-adjusting networks: networks which are optimized towards, and "match", the traffic workload they serve. We will discuss information-theoretic metrics to quantify the structure in communication traffic as well as the achievable performance in datacenter networks matching their demands, present network design principles accordingly, and identify open research challenges. I will also show how the notions of self-adjusting networks and demand-aware graphs relate to classic optimization problems in theoretical computer science.
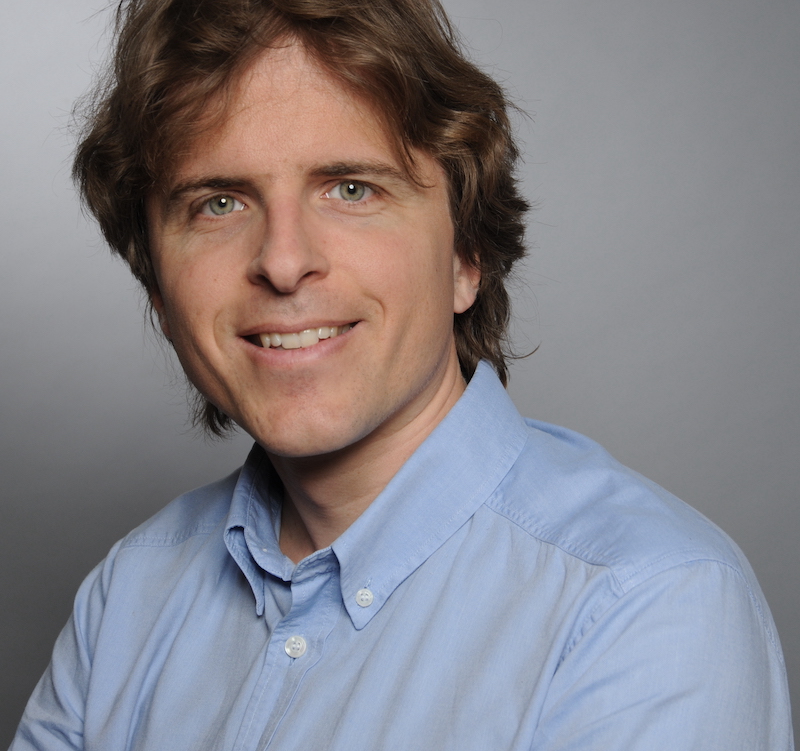
Since 2021, he is a Council and Board member of the European Association of Theoretical Computer Science (EATCS) and also serves as the Editor-in-Chief of the Bulletin of the EATCS. Since 2019 Stefan Schmid is an Editor of IEEE/ACM Transactions on Networking (ToN). From 2015 to 2021, he was the Editor of the Distributed Computing Column of the Bulletin of the EATCS, and from 2016 to 2019, an Associate Editor of IEEE Transactions on Network and Service Management (TNSM). Stefan Schmid received the IEEE Communications Society ITC Early Career Award 2016 and acquired several major grants including an ERC Consolidator Grant, various other EU grants (e.g., STREP and IP projects) and national grants (e.g., three FWF projects), a German-Israeli GIF grant, a Villum Fonden grant, a WWTF grant, and various German grants (e.g., from BSI and BMBF). In 2015, he co-founded the startup company Stacktile supported by Germany's EXIST program, and in 2020, he helped establish the Vienna Cybersecurity and Privacy Research Center (ViSP) for which he also served in the executive board. Stefan Schmid's research interests revolve around the fundamental and algorithmic problems of networked and distributed systems.
Homepage
Next-generation Networks for Machine Learning
Abstract: The ever-growing demand for more accurate machine learning models has resulted in a steady increase in dataset and model sizes of deep neural networks (DNNs). Although hardware accelerators have provided a significant amount of speed-up, today's DNN models can still take days and even weeks to train mainly because conventional datacenter networks are becoming a bottleneck for distributed DNN training workloads. In this talk, I will discuss two techniques to accelerate DNN training workloads. First, I will present a novel optical fabric that co-optimizes the network topology and parallelization strategy for DNN clusters. Second, I will argue that fair-sharing, the holy grail of congestion control algorithms for decades, is not necessarily a desirable property in DNN training clusters and propose a scheduling technique that carefully places jobs on network links to avoid bandwidth sharing.
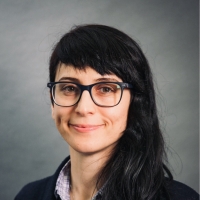
Homepage
Heterogeneous Serverless Computing
Abstract: The high performance computing is evolving rapidly, shaped by the confluence of three trends: a) traditional simulation and modeling workloads are converging with massive data analytic and AI/ML workflows; b) the efficiency of special purpose heterogeneous hardware is increasing; and c) the demand for flexible delivery models that blend traditional on-premises deployments with cloud-like as-a-service models continues to grow. Heterogeneity is driven by the end of Moore's Law, growth of data, and by the emergence of broad AI adoption that is well-suited for special-purpose hardware. To date, serverless computing abstracts the complexity of the underlying infrastructure by leveraging homogeneity and is motivated by simplified DevOps experience for new composable and scalable applications. Delivering the efficiency of heterogeneity, the productivity of serverless, and the granularity of Functions-as-a-Service demands a new architecture.
The Heterogeneous Serverless Computing (HSC) aims to enable development and delivery of HPC, HPDA, and AI (H2A) workloads with the ease and efficiency of the Cloud and with higher scale and more fluidity than supercomputers. HSC is a software-hardware co-designed infrastructure supporting H2A workflow execution economically and securely at fine granularity using Functions as a Service (FaaS). HSC targets the changeover evolution to H2A workflows with flexible consumption models, the edge-to-exascale deployment, and embraces a more maintainable, scalable, and re-usable development model. We focus on innovative uses of accelerators, such as in SmartNICs and Fabric Attached Memories, to improve performance of H2A applications and efficiency of hardware, but without compromising ease of development.
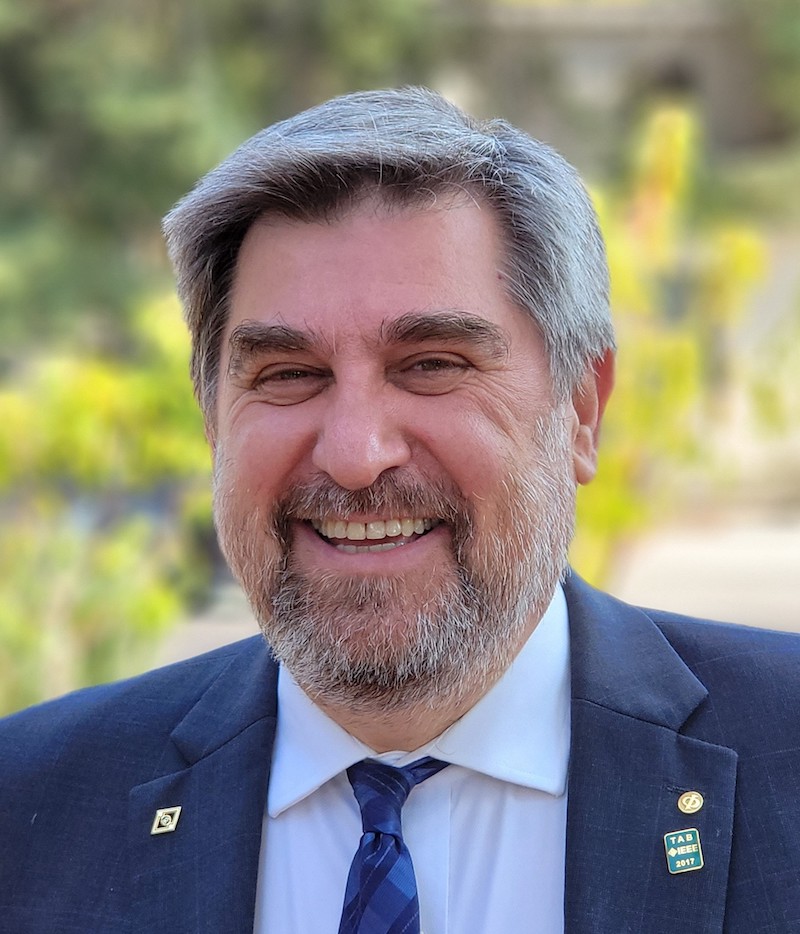
Dejan was president of the IEEE Computer Society, IEEE presidential candidate, editor-in-chief of IEEE Computing Now and IEEE Distributed Systems Online and he has served and continues to serve on many editorial boards, technical program committees and steering committees. Previously, Dejan worked in the OSF Research Institute, Cambridge, MA and Institute "Mihajlo Pupin", Belgrade, Serbia. He contributed to novel systems software and parallel and distributed systems that were deployed throughout Europe. Dejan received his Ph.D. from the University of Kaiserslautern, Germany; and his MSc/BSc from Belgrade University, Serbia.
Homepage
AI Engine Architecture: Data Movement, Synchronization, Reconfiguration & Application Mapping
Abstract: AI Engine (AIE) is an array of vector processors developed by AMD/Xilinx. AIE is part of both the Xilinx Versal 7nm family of devices and next-gen AMD APU devices. This architecture is composed of a 2D array of tiles, where each compute tile includes a VLIW SIMD vector processor, scratchpad memory, data movement engines and streaming interconnect. Target applications include machine learning inference in datacentre, automotive and edge, as well as wireless (5G) acceleration. In this talk, David will present an overview of the architecture and then go into details on data movement, synchronization, reconfiguration, and application mapping onto hardware.
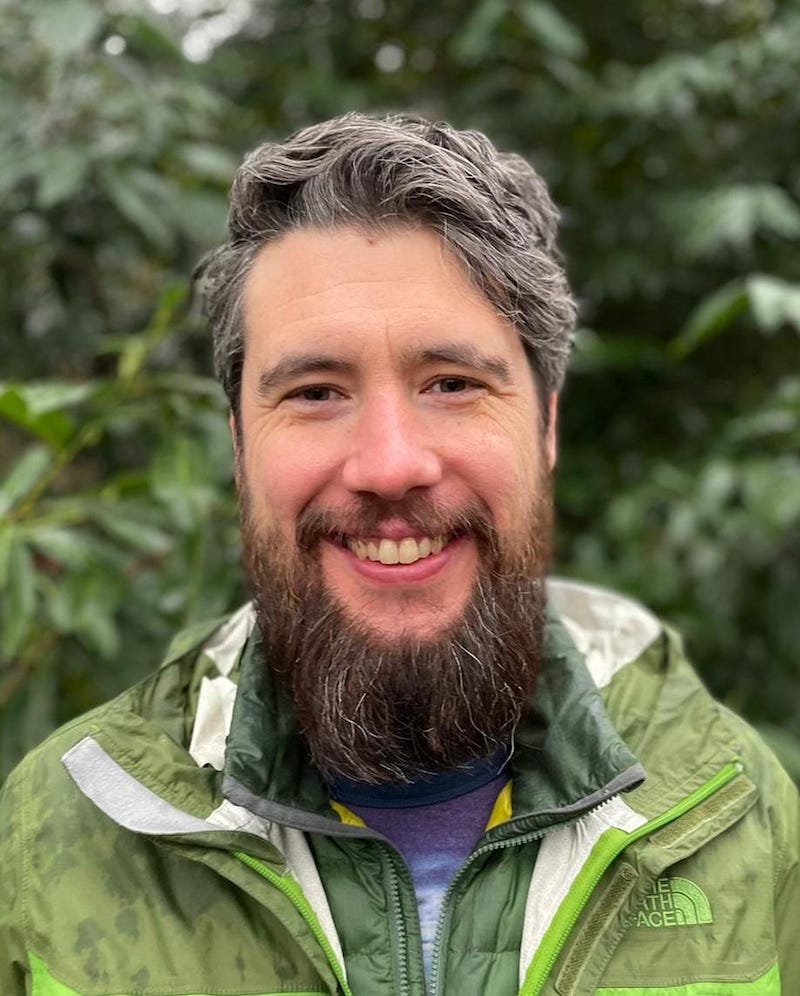
Homepage
Innovating the Next Discontinuity
Abstract: A growing number of classical HPC applications - modeling and simulation applications - are bottlenecked due to insufficient memory bandwidth. At the same time, AI applications, which are forming an increasingly important part of HPC, and compute in general, are often bottlenecked because of insufficient communication (node to node) bandwidth. In addition, the ability to leverage efficient accelerator cycles for both types of applications is key towards continuing the exponential growth for post-exascale computing. In this talk I will describe the key trends identified above, and discuss the research we are undertaking to design the hardware and software architecture for HPC and AI applications to obtain the next level of exponential increase in performance. I will suggest a path forward based on leveraging tightly integrating memory and compute, called Memory Couple Compute, and describe the interesting design space that needs to be considered to make this architecture a reality. This capability has the potential to be the next discontinuity in HPC and AI.
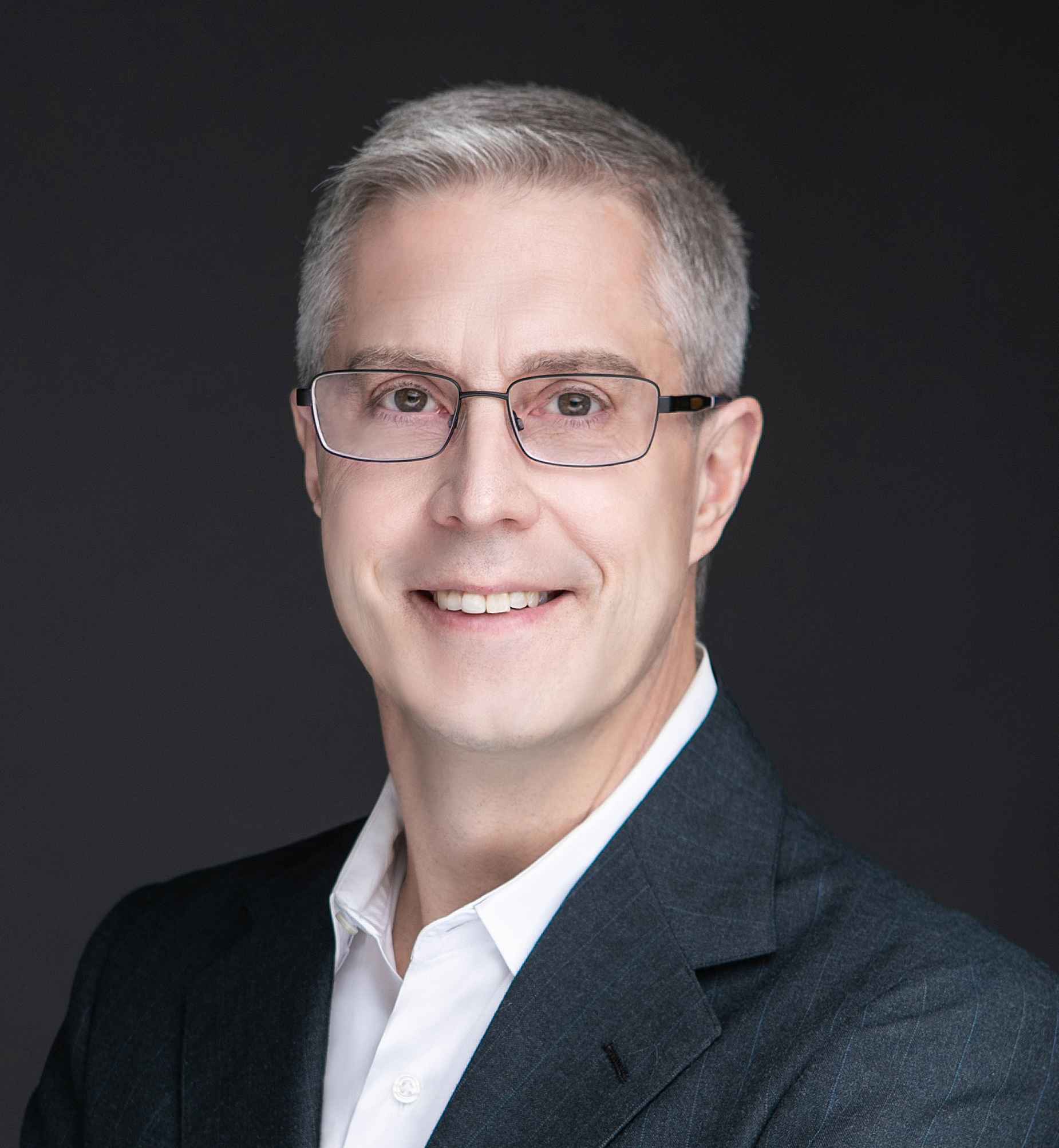
Homepage
Democratizing Deep Learning with DeepHyper
Abstract: Scientific data sets are diverse and often require data-set-specific deep neural network (DNN) models. Nevertheless, designing high-performing DNN architecture for a given data set is an expert-driven, time-consuming, trial-and-error manual task. To that end, we have developed DeepHyper [1], a software package that uses scalable neural architecture and hyperparameter search to automate the design and development of DNN models for scientific and engineering applications. In this talk, we will present our recent work on an automated approach for generating an ensemble of DNNs with DeepHyper at scale and using them for estimating data (aleatoric) and model (epistemic) uncertainties for a wide range of scientific applications.
[1] DeepHyper
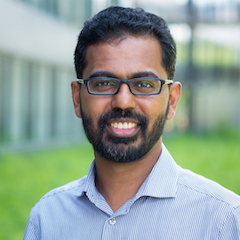
Homepage
Follow the Data: Memory-Centric Designs for Modern Datacenters
Abstract: Memory has passed compute as the most critical determiner of system performance, as well as the largest component of cost. However, decisions about memory architecture are often left as an afterthought or decided by "rules of thumb" or "zeitgeist" instead of the quantitative/analytical approaches common in computer architecture.
In this talk, data-driven approaches for setting direction in memory architectures will be explored through the lens of two different system-level memory problems: Exascale supercomputing and Cloud memory disaggregation.
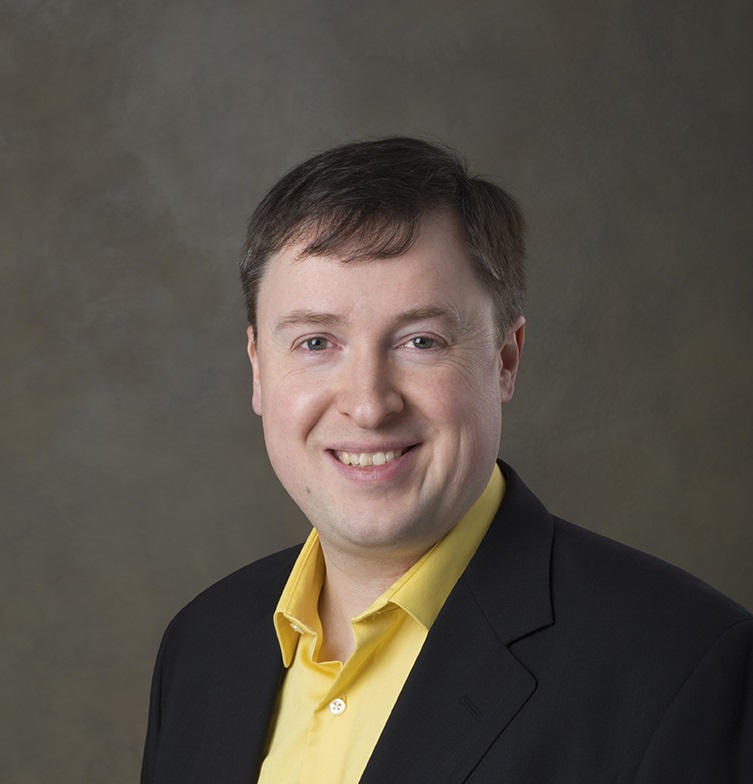
Homepage
HPC and AI/ML: A Synergistic Relationship
Abstract: The rapid increase in memory capacity and computational power of modern architectures, especially accelerators, in large data centers and supercomputers has led to a frenzy in training extremely large deep neural networks. However, efficient use of large parallel resources for extreme-scale deep learning requires scalable algorithms coupled with high-performing implementations on such machines. In this talk, I will first present AxoNN, a parallel deep learning framework that exploits asynchrony and message-driven execution to optimize work scheduling and communication, which are often critical bottlenecks in achieving high performance. I will also discuss how neural network properties can be exploited for different systems-focused optimizations. On the other hand, recent advances in machine learning approaches are driving scientific discovery across many disciplines, including computer systems and high performance computing. AI/ML can be used to explore the vast quantities of system monitoring data being collected on HPC systems. I will also present a few examples of using data-driven ML models for performance modeling, forecasting and code generation to highlight how the fields of HPC and AI/ML are coming together, and can help each other.
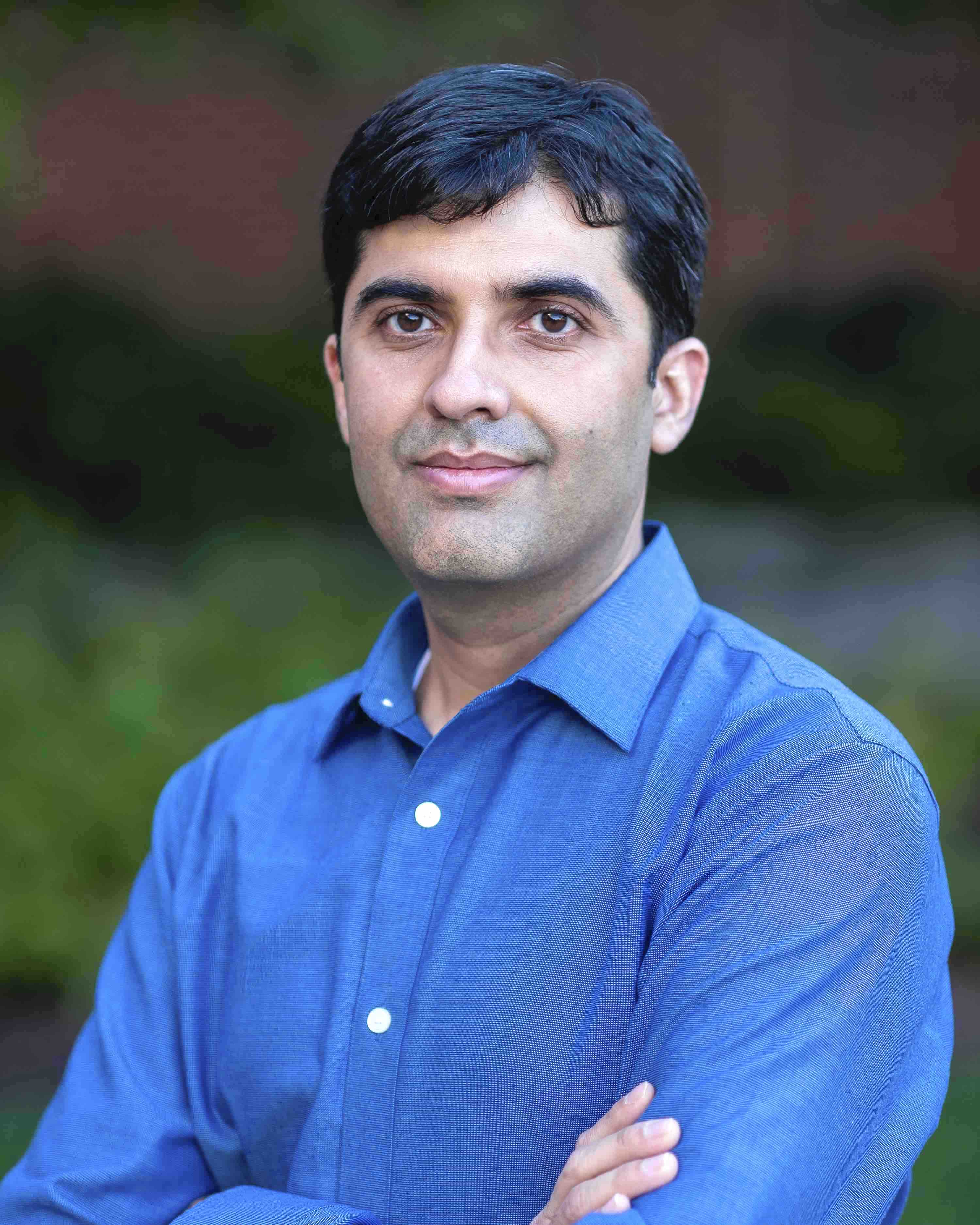
Homepage
A chiplet based generative inference architecture with block floating point datatypes
Abstract: The advent of large transformer based language models (BERT, GPT3, ChatGPT, Lamda, Switch) for Natural Language Processing (NLP) and their growing explosive use across Generative AI business and consumer applications has made it imperative for AI accelerated computing solutions to provide an order of magnitude improvements in efficiency. We will discuss a modular, chiplet based spatial CGRA-like architecture optimized for generative inference with a generalized framework for the successful implementation of deep RL-based mappers in compilers for spatial and temporal architectures. We’ll present results for weight and activation quantization in block floating point formats, building on GPTQ and SmoothQuant, and their support in PyTorch. To reduce KV cache size and bandwidth, we’ll present an extension to EL-attention.
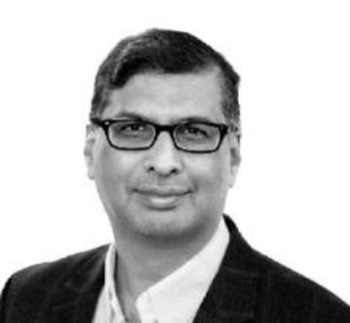
Homepage
Realizing Petabit/s IO and sub-pJ/bit System-wide Communication with Silicon Photonics
Abstract: High-performance systems are increasingly bottlenecked by the energy and communications costs of interconnecting numerous compute and memory resources. Integrated silicon photonics offer the opportunity of embedding optical connectivity that directly delivers high off-chip communication bandwidth densities with low power consumption. Our recent work has shown how integrated silicon photonics with comb-driven dense wavelength-division multiplexing can scale to realize Pb/s chip escape bandwidths with sub-picojoule/bit energy consumption. Beyond alleviating the bandwidth/energy bottlenecks, embedded photonics can enable new architectures that leverage the distance independence of optical transmission with flexible connectivity tailored to accelerate distributed ML applications.
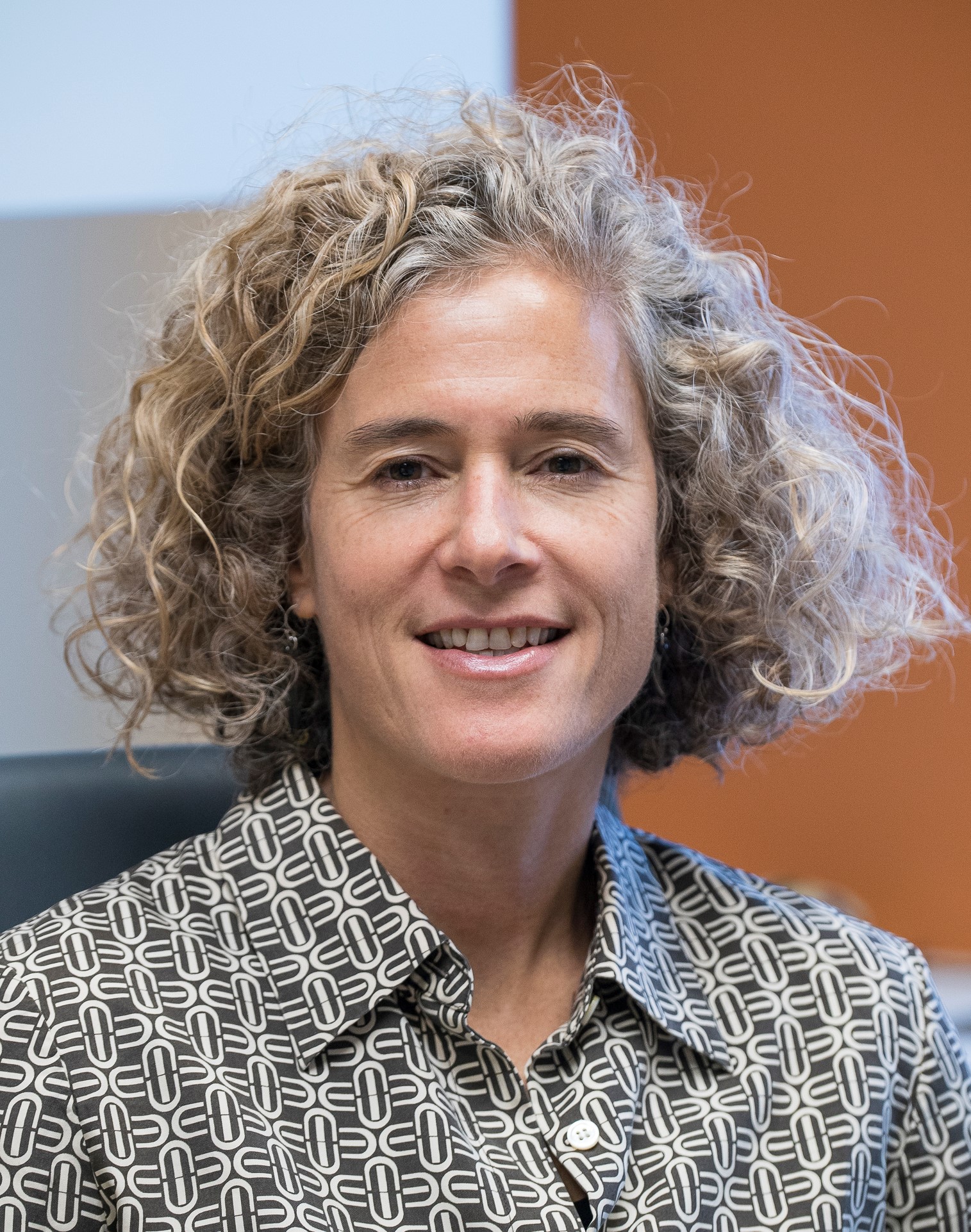
Homepage
HPVM: Performance, Programmability and Retargetability for Heterogeneous Parallel Systems
Abstract: Heterogeneous parallel systems are becoming increasingly prevalent in today's mobile devices and low-energy edge computing products, like smart cameras, mobile robots, AR/VR headsets, and others. These heterogeneous systems deliver orders-of-magnitude power-performance benefits compared with multicore CPUs, but are notoriously difficult to program, even for computing experts. Moreover, the diverse and fast-evolving instruction sets for both CPUs with complex vector, matrix and tensor architectures and for specialized accelerators are difficult to target from retargetable compiler systems like LLVM and GCC. The broad goal of the Heterogeneous Parallel Virtual Machine (HPVM) project is to enable both expert and non-expert application developers to be able to program heterogeneous parallel systems while achieving good performance and remaining no more difficulut to program than traditional parallel systems. HPVM is a highly retargetable compiler infrastructure that can compile different parallel languages to a wide range of hardware targets, including diverse CPUs, GPUs, FPGAs, fixed-function accelerators, and programmable machine learning accelerators. In this talk, I will describe two broad aspects of the HPVM project. The first is on enabling ``hardware-agnostic programming'' (a term we will explain more carefully) with good performance on diverse heterogeneous hardware targets, by using a combination of compiler optimizations, autotuning and design space exploration. The second is on automatically generating highly retargetable, yet very high performance, code generators for vector and matrix architectures. Given the vendor-defined pseudocode specification of one or more target ISAs, we automatically generate AutoLLVM IR, which consists of (formally defined) language-independent and target-independent LLVM IR instructions to support those ISAs. A Halide language compiler implemented fully automatically using AutoLLVM for both x86+AVX-512 and HVX, given only a formal semantics of the Halide front-end IR, is able to outperform a mature, well-tuned production compiler for Halide on both X86 and HVX across a wide range of benchmarks.
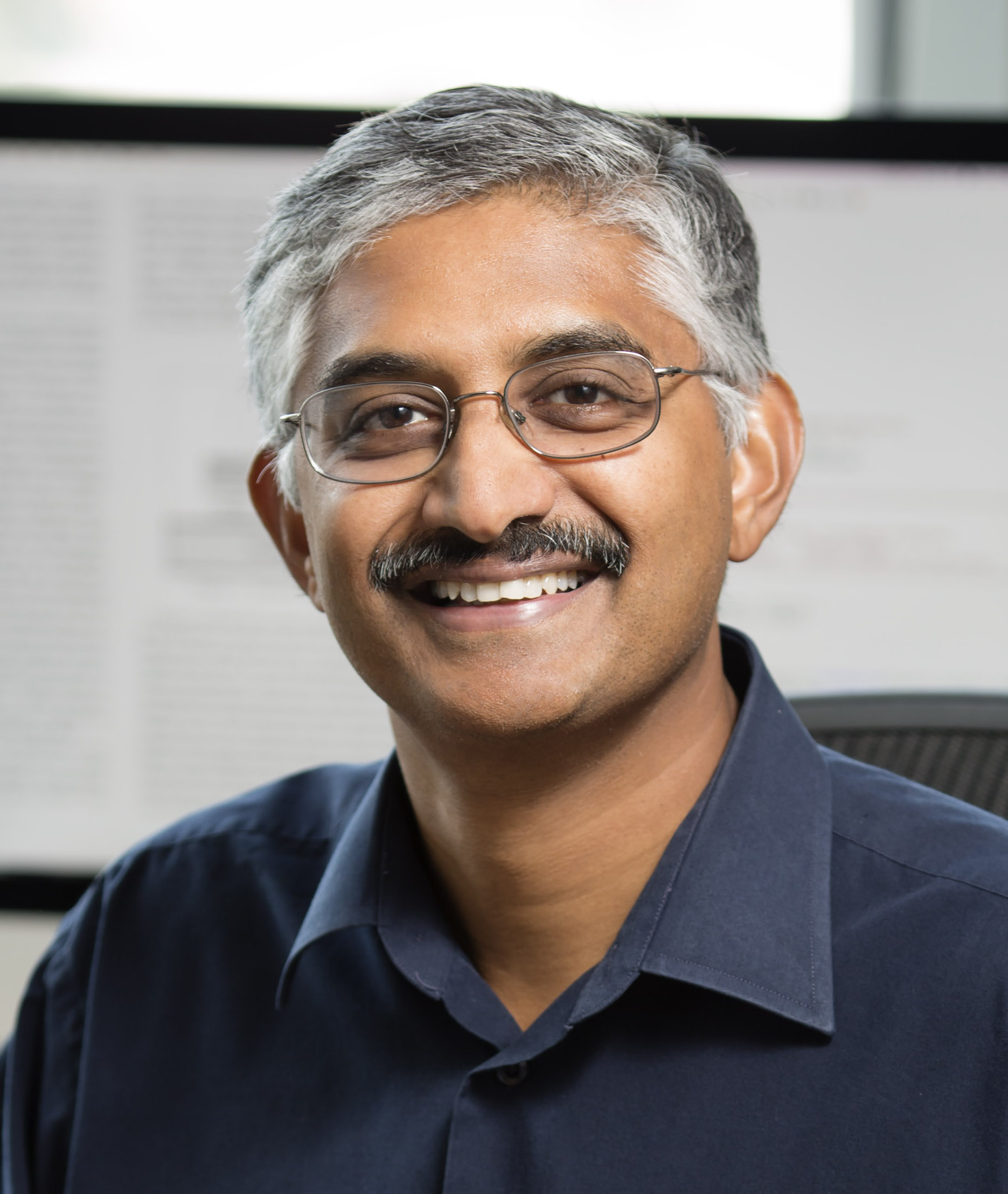
Homepage
Behind the Pixels: Challenges of Scaling and Optimizing Infrastructure for Animation Workloads
Abstract: Making an animated feature film is no small feat. At Walt Disney Animation Studios, hundreds of artists and engineers collaborate together for years to tell stories that delight audiences around the world. Each year, films grow in complexity as artists push the boundaries of what had been impossible just the year before. In this talk I will discuss some of the challenges we’re working on in our datacenter as we continue to try to make the impossible possible. First, I will look at challenges related to storage, like how to identify and squash the workloads on our renderfarm that consistently generate the NFS metadata operations that account for 95% of the activity on our file server. Next, I will talk about challenges on our renderfarm as we seek to improve the utilization of every CPU core and gigabyte of DRAM in the facility. This includes weighing the pros and cons of hyperconverged filesystems and disaggregated memory. Lastly, I will talk about where storage meets the renderfarm and discuss challenges around making data available to artists at their desktops while also simultaneously making that same data available to a remote renderfarm in the public cloud; all while allowing either side to make modifications at any time.
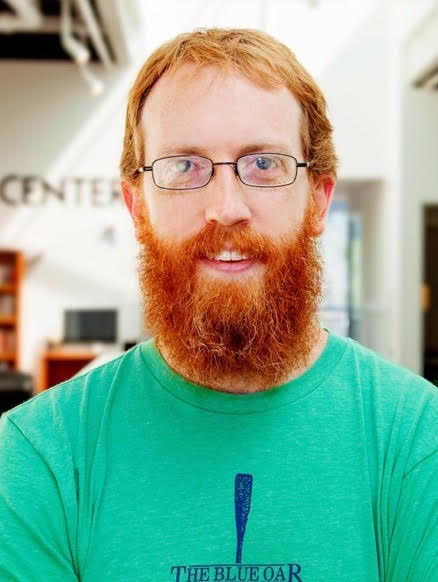
Homepage
Evaluating Large-Scale Learning Systems
Abstract: To deploy machine learning models in practice it is critical to have a way to reliably evaluate their effectiveness. Unfortunately, the scale and complexity of modern machine learning systems makes it difficult to provide faithful evaluations and gauge performance across potential deployment scenarios. In this talk I discuss our work addressing challenges in large-scale ML evaluation. First, I explore the problem of evaluating models trained in federated networks of devices, where issues of device subsampling, heterogeneity, and privacy can introduce noise in the evaluation process and make it challenging to provide reliable evaluations. Second, I present ReLM, a system for validating and querying large language models (LLMs). Although LLMs have been touted for their ability to generate natural-sounding text, there is a growing need to evaluate the behavior of LLMs in light of issues such as data memorization, bias, and inappropriate language. ReLM poses LLM validation queries as regular expressions to enable faster and more effective LLM evaluation.
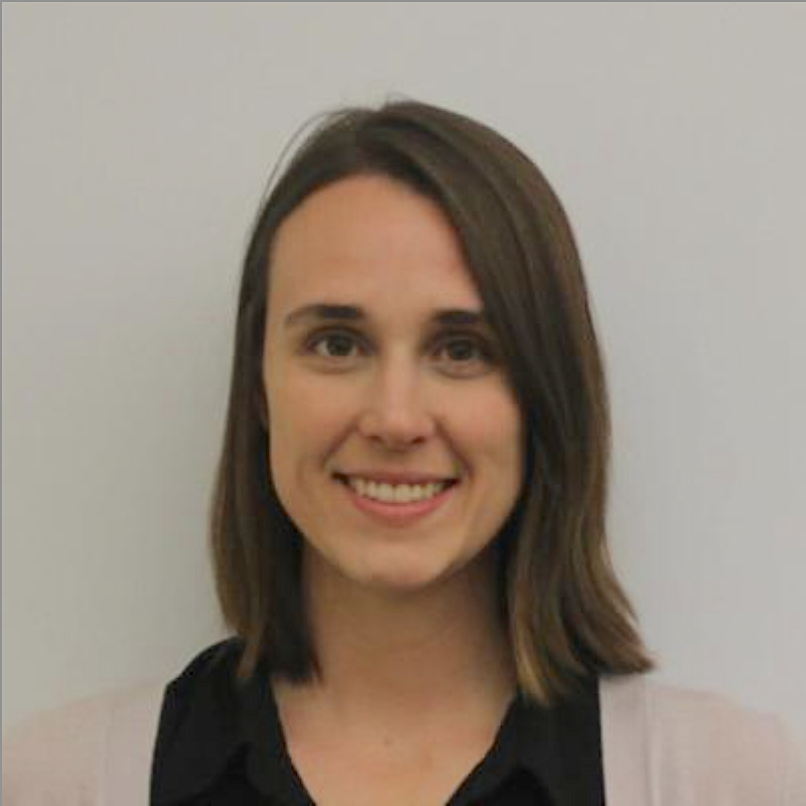
Homepage
Heterogeneous multi-core systems for efficient EdgeML
Abstract: Embedded ML applications are characterized by increasingly diverse workloads, forming a rich mixture of signal processing, GeMM and conv kernels, attention layers, and even graph processing. Accelerator efficiency suffers from supporting this wide variety of kernels. Heterogeneous multicore systems can offer a solution but come with their own challenges, such as: 1.) How to find the most optimal combination of cores?; 2.) How to efficiently map workloads across cores?; 3.) How to share data between these cores? This talk will report on a heterogeneous multi-core system for embedded neural network processing taped out at KULeuven MICAS. Moreover, it will give an outlook on work in progress towards further expanding this system for covering more workloads and more heterogeneous cores.
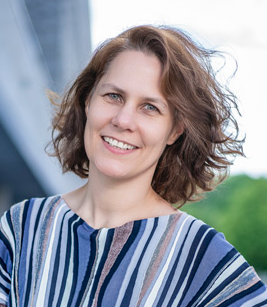
Homepage
Scalable Graph Machine Learning
Abstract: Recently, Graph Neural Networks (GNNs) have been used in many applications leading to improved accuracy and fast approximate solutions. Training as well as Inference in these networks is computationally demanding. Challenges include access to irregular data, large scale sparse as well as dense matrix computations, limited data reuse and heterogeneity in the various stages of the computation. This talk will review our recent work in the Data Science Lab (dslab.usc.edu) and FPGA/Parallel Computing Lab (fpga.usc.edu) at USC leading up to current trends in accelerators for data science. For graph embedding, we develop GraphSAINT, a novel computationally efficient technique using graph sampling and demonstrate scalable performance. We develop graph processing over partitions (GPOP) methodology to handle large scale graphs on parallel platforms. On a current FPGA device, we demonstrate up to 100X and 30X speed up for full graph GNN computations compared with state-of-the-art implementations on CPU and GPU respectively. We also demonstrate specific accelerators for two widely used GNN models: GraphSAGE and GraphSAINT. We conclude by identifying opportunities and challenges in exploiting emerging heterogeneous architectures towards a general framework for GNN acceleration.
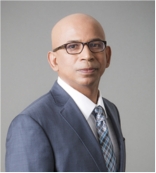
Homepage
Can I Cook a 5 o'clock Compiler Cake and Eat It at 2?
Abstract: In high-performance computing words: can we build a compiler that will eventually save a lot of performance engineering effort while immediately delivering competitive results? Here, competitiveness refers to achieving near hardware peak-performance for important applications. The question is particularly hot in a domain-specific setting, where the building blocks for constructing an effective optimizing compiler may be inadequate, too generic, or too low-level. It is widely understood that compiler construction has failed to deliver early afternoon sweets. I personally feel bad about it, but until recently it remained an academic exercise to challenge the status quo. Maybe it is now time to reconsider this assumption: ML-enhanced compilers become the norm rather than the exception. New compiler frameworks reconcile optimizations for the common case with application-specific performance. Domain-specific code generators play an essential role in the implementation of dense and sparse numerical libraries. But even with the help of domain-specific compilers, peak performance can only be achieved at the expense of a dramatic loss of programmability. Are we ever going to find a way out of this programmability/performance dilemma? What about the velocity and agility of compiler engineers? Can we make ML-based heuristics scalable enough to compile billions of lines of code? Can we do so while enabling massive code reuse across domains, languages and hardware? We will review these questions, based on recent successes and half-successes in academia and industry. We will also form an invitation to tackle these challenges in future research and software development.
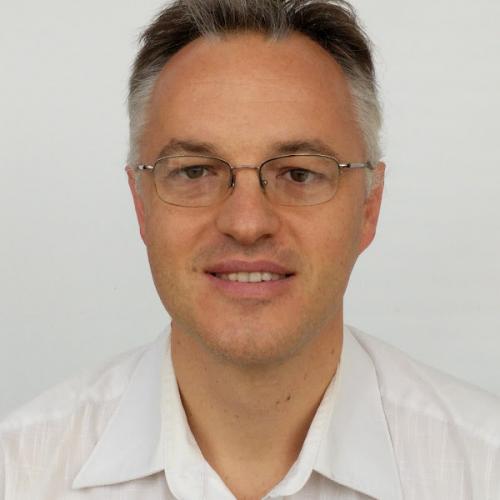
Homepage
Capturing Computation with Algorithmic Alignment
Abstract: What makes a neural network better, or worse, at fitting certain tasks? This question is arguably at the heart of neural network architecture design, and it is remarkably hard to answer rigorously. Over the past few years, there have been a plethora of attempts, using various facets of advanced mathematics, to answer this question under various assumptions. One of the most successful directions -- algorithmic alignment -- assumes that the target function, and a mechanism for computing it, are completely well-defined and known (i.e. the target is to learn to execute an algorithm). In this setting, fitting a task is equated to capturing the computations of an algorithm, inviting analyses from diverse branches of mathematics and computer science. I will present some of my personal favourite works in algorithmic alignment, along with their implications for building intelligent systems of the future.
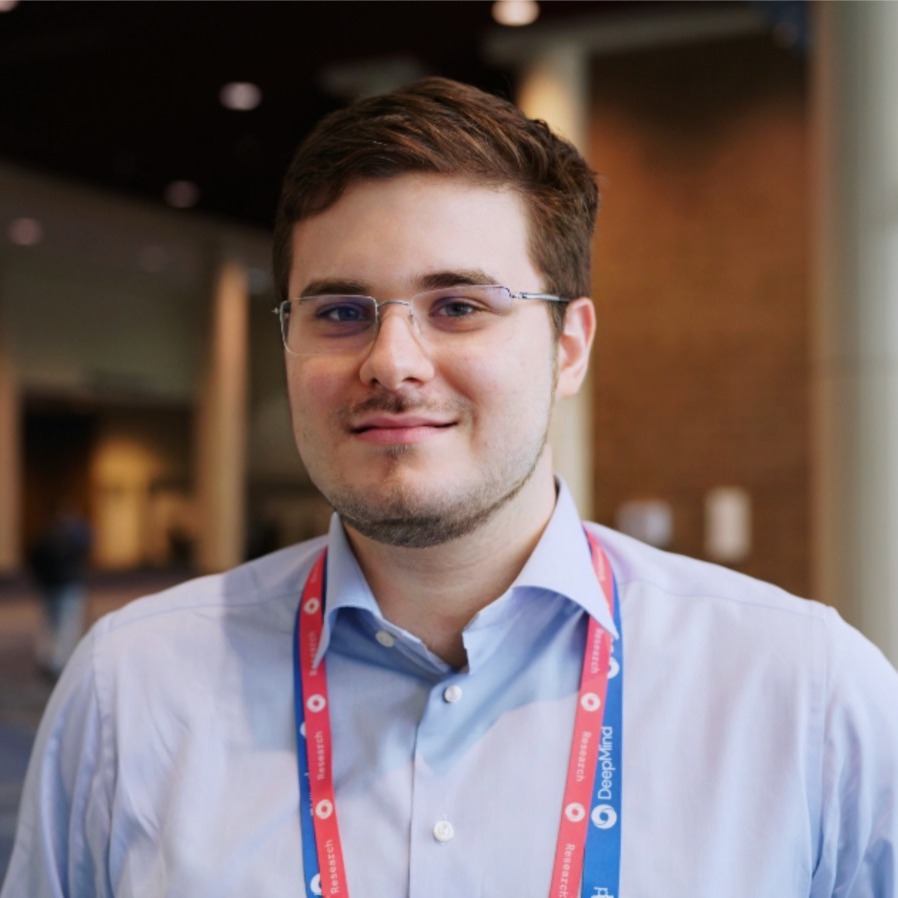
Homepage
The digital revolution of Earth system modelling
Abstract: This talk will outline three revolutions that happened in Earth system modelling in the past decades. The quiet revolution has leveraged better observations and more compute power to allow for constant improvements of prediction quality of the last decades, the digital revolution has enabled us to perform km-scale simulations on modern supercomputers that further increase the quality of our models, and the machine learning revolution has now shown that machine learned weather models are often competitive with conventional weather models for many forecast scores while being easier, smaller and cheaper. This talk will summarize the past developments, explain current challenges and opportunities, and outline how the future of Earth system modelling will look like.
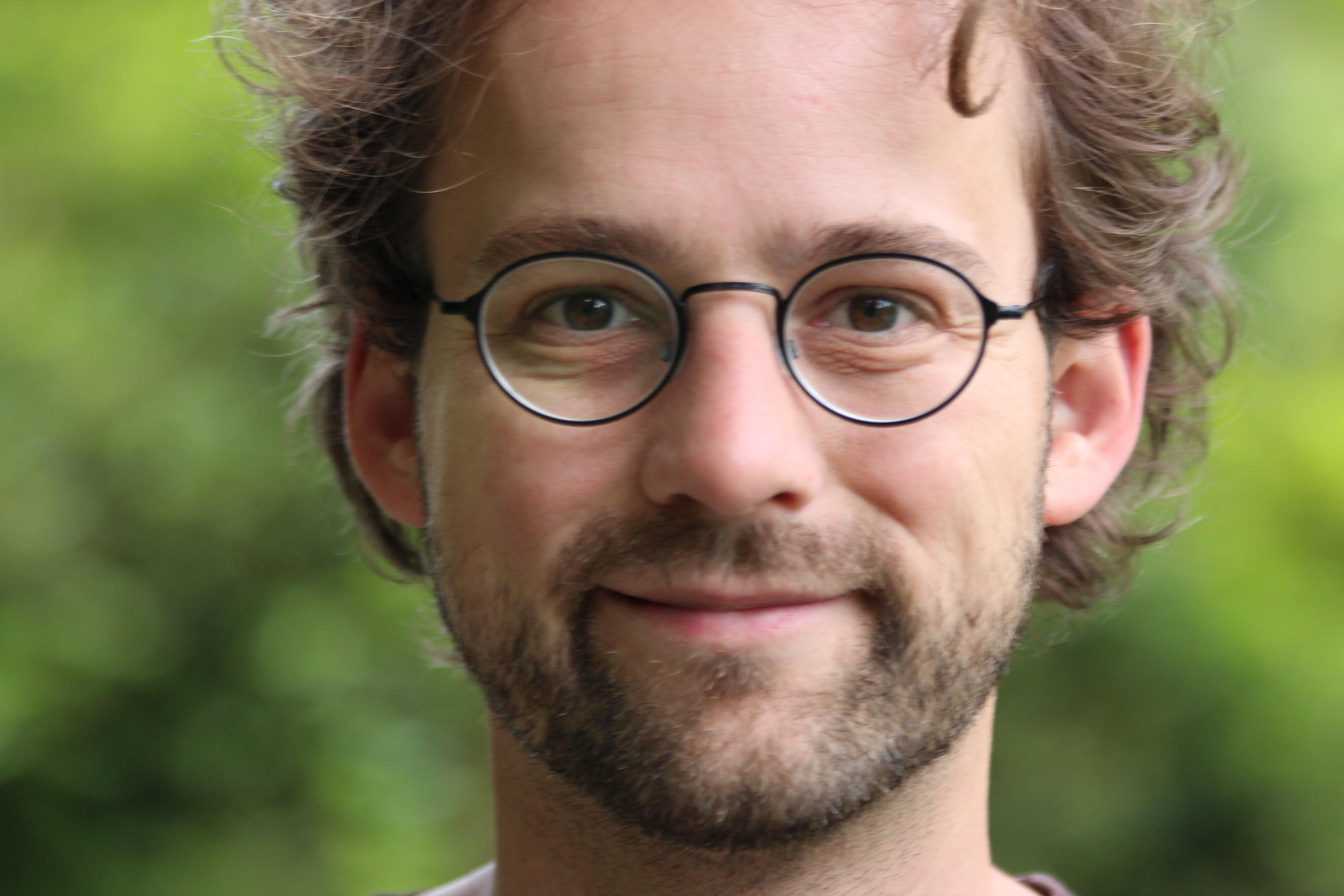
Homepage
Improving Cloud Security with Hardware Memory Capabilities
Abstract: More and more data-intensive applications, e.g., micro-service architectures and machine learning workloads, move from on-premise deployments to the cloud. Traditional cloud security mechanisms focus on strict isolation, but applications also require the efficient yet secure sharing of data between components and services. In this talk, I will explore how we can use a new hardware security feature, memory capabilities, to design a cloud stack that bridges the tension between isolation and sharing. Memory capabilities constrain memory accesses, and they can be used to provide a VM-like isolation mechanism, cVMs, that can share data more efficiently than containers. They can also increase memory efficiency by safely de-duplicating application components. I will discuss our experience in building a cloud stack with memory capabilities on the CHERI architecture, as implemented by Arm’s Morello hardware.
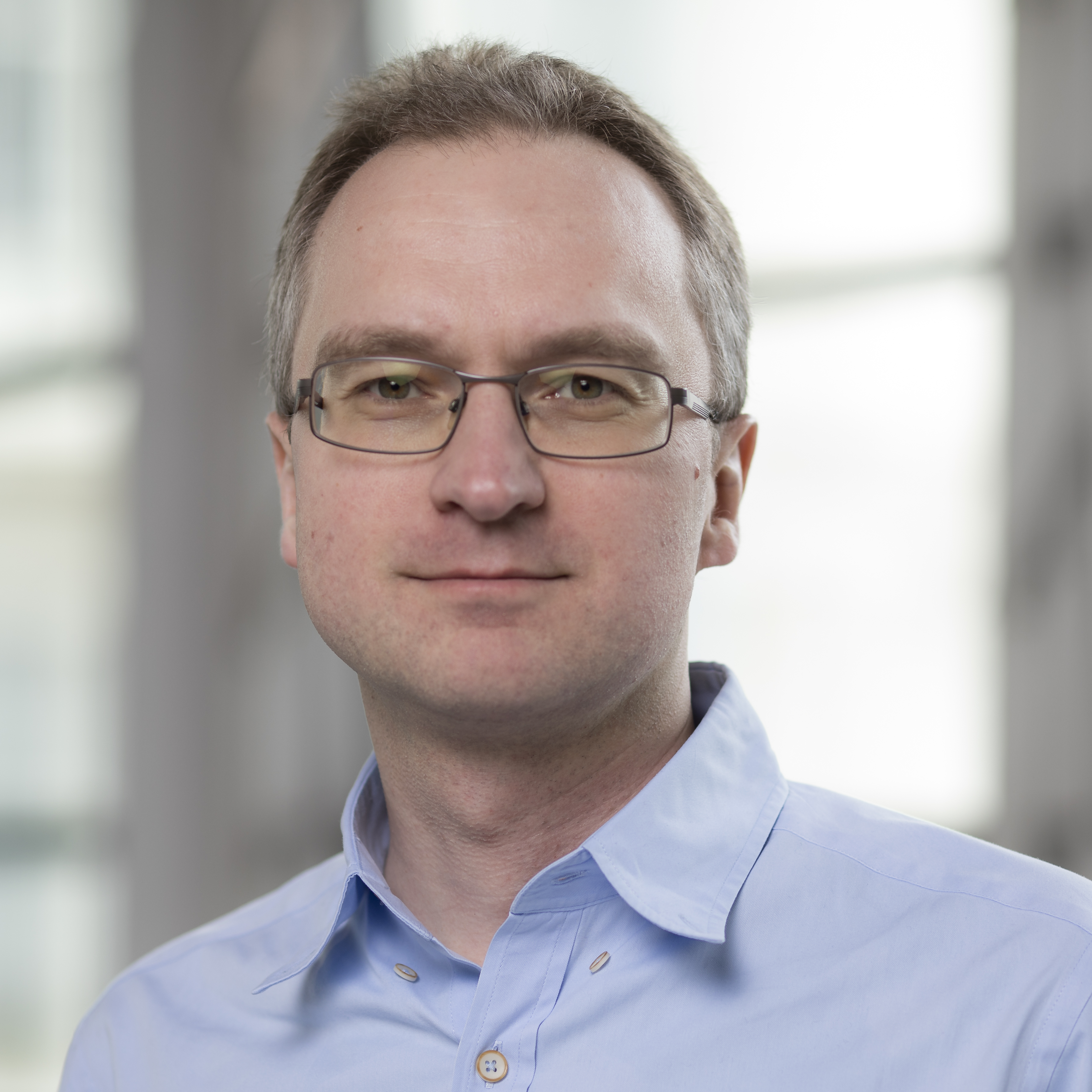
Homepage